Giving AI Some Common Sense: A Q&A with Ron Brachman (Pt. 1)
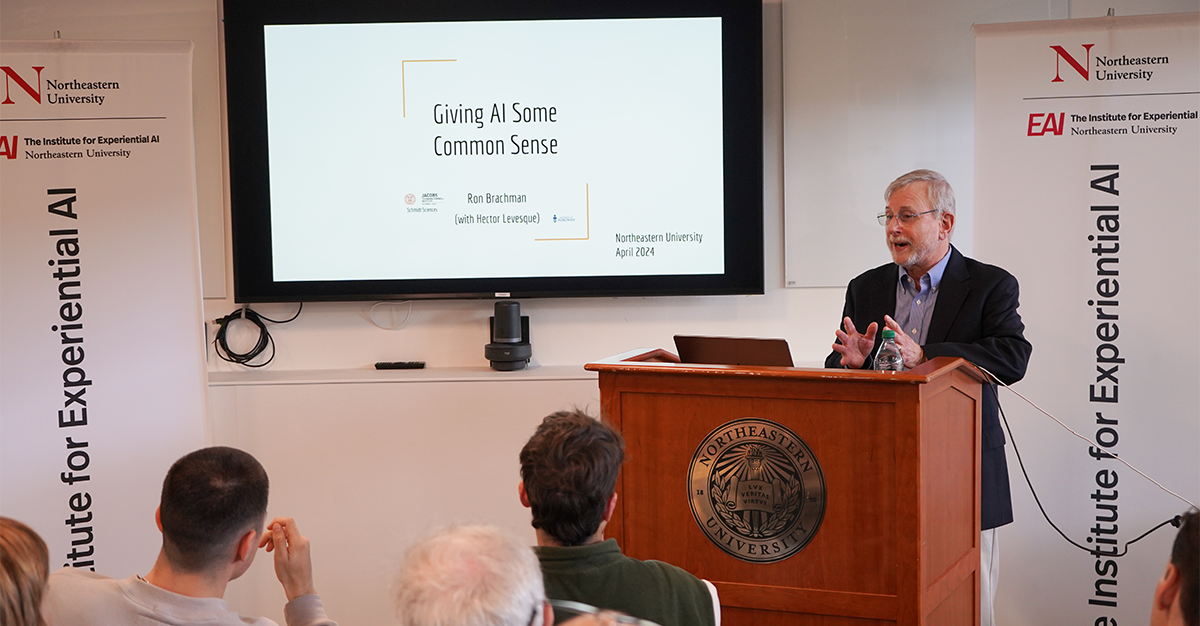
Does AI have common sense? Most experts say no. But can it be made to have common sense? For Ron Brachman, answering that question means going back to the drawing board, and perhaps getting a little philosophical about what common sense even means..
Brachman is the director of the Jacobs Technion-Cornell Institute at Cornell Tech, and as part of a Distinguished Lecturer Seminar hosted by the Institute for Experiential AI, he made the case that common sense is all of the following: broadly known, obvious, simple, based on experience, practical, and generally about mundane things.
Following the talk, he was kind enough to answer questions from audience members about relativism in common sense reasoning, responsibility in autonomous systems, and the limits of computer science. You can find the second part of the Q&A here.
1. A large amount of common sense is unconscious. How do you factor in the unconscious when it comes to machines and AI? Do they have an unconscious?
We humans are not consciously aware of much of what we do in everyday life, and in that regard common sense is no different than other kinds of mental activities. (Of course we are awake and conscious while we’re doing everyday activities — we can’t do them if we are unconscious in the normal sense of the word.) We can stop and reflect on our desires, decisions, and actions, but I am not sure I see a difference in doing that for activities that are commonsensical and those that are more deeply analytical and methodical. We are certainly aware when using either kind of reasoning facility (as opposed to rote, repetitive, habitual activity, for which we might argue we don’t have to do any kind of “thinking” to be operating). In many (most?) cases of what we do every day, we are not directly aware of the computations behind our decisions, but as I mentioned in the talk, both common sense and more rigorous or expert analysis can be influenced by new observations or verbal guidance (what I was calling “cognitive penetrability”). But even then, we may not be aware of how those external influences change our behavior.
There is no general agreement on the exact role that consciousness plays or where it comes from. It is in some ways a mysterious quality — we probably can’t even prove that we’re conscious. I don’t think anyone would argue that current AI systems have anything resembling consciousness, but that doesn’t stop such systems from displaying what appears to be intelligent behavior. So one might argue that as of now, everything AI does is “unconscious,” but it’s unclear whether that is significant in any way.
2. What evidence warrants confidence that common sense reasoning — or any other prerequisite to AGI (Artificial General Intelligence) — is ultimately achievable within the scope of computer science?
I don’t think there is anything magic or ineffable about commonsense reasoning, and through the history of AI there have been many efforts that you could argue have achieved it in limited situations. The examples I gave in the talk, from Cyc and ChatGPT, look to me like in certain limited ways AI systems have already demonstrated commonsense reasoning. (We could argue about whether LLMs actually do “reasoning,” but I don’t think that is the point of your question — they are certainly performing computations.) There are also several benchmarks (e.g., CommonsenseQA, BIG-bench) that cover some aspects of commonsense reasoning, and some systems have done modestly well on these.
While the talk pointed out that so far most of this work has focused on piecemeal, transactional interactions with commonsense “knowledge bases” (what I likened to “fact calculators”), I think there is a path to using those capabilities in a more comprehensive cognitive system, which would comprise a couple of components carrying out different responsibilities, and sharing information among them in a way that could ultimately result in a pretty convincing display of end-to-end commonsensical behavior in an AI system (this is what I illustrated in my architecture slide). I haven’t seen anything written about common sense that would suggest that it would not be amenable at some point to computational analysis and implementation in a machine. We may need to continue to invent new inference regimes (more relaxed than pure deductive logic), but progress has been made on such things and I don’t see a fundamental roadblock to an eventual comprehensive computer science analysis.
3. Will deep neural networks ever achieve common sense?
It’s of course almost impossible to answer a question that asks about “ever,” but in my view, the current ML-based architectures that are prevalent in AI will need some augmentation to go beyond the piecemeal capabilities to answer common sense questions they currently display. As you may be aware, there are shortcomings in these systems related to reasoning, arithmetic, planning, and other cognitive functions that more classical AI is capable of handling, and I do think these will play a role in achieving true end-to-end common sense of the sort I discussed in my talk. Some of the very large-scale neural-net based systems already display some pretty convincingly commonsensical answers to queries. But as I tried to emphasize, common sense as a whole is really about overall sensible behavior, which may involve many small commonsense fact retrievals, but those have to be woven together based on the system’s goals and desires in a rational way, often based on a strong understanding of causality and the usual effects of actions.
To my mind, it’s not inconceivable that a purely neural-net-based system could achieve this overall behavior, but as currently implemented it feels like they will need augmentations that may use different technology. Furthermore, while perhaps not directly a part of “common sense,” the kind of ability I talked about whereby a system could adopt advice or change its beliefs based on situational input will need the ability to isolate the specific beliefs about which the advice has been given, and it’s hard to see how that would be done in the current style of network architectures. But that doesn’t mean it would never be possible with some technology evolution and new research.
4. This is related to your comment on the benefits of relying on principles in combination to probabilities to get closer to common sense. I am trying to figure out how AI can recapitulate human scientific evolution by deriving principles from large observable data sets. Do you think this makes sense?
This is an important point. Very young children, through empirical trial-and-error interaction, gradually acquire generalities about the world. Arguably, this is done through data-driven learning, and the volume of data accessed by children through their senses and via manipulation is very, very large. Eventually, these coalesce somehow into the kind of principles we’re talking about, which can eventually be built upon through instruction in school and verbal teaching. If you’re interested in this, the DARPA Machine Common Sense program is worth a look; it worked to bring together AI people doing work on more classical (e.g., symbolic-reasoning-based) commonsense reasoning with psychologists who understood infant development. There is very interesting work done in that context by many people, including Josh Tenenbaum and his group at MIT and Allison Gopnik and colleagues at Berkeley (this, for example).
5. Could we use LLMs to construct knowledge graphs automatically?
To an extent, yes, although there is currently concern about the accuracy/validity of the knowledge extracted from LLMs. There is already some interesting work along these lines that you may want to investigate. For example, what has been called “symbolic knowledge distillation” has been pursued by Yejin Choi and others (see, for example, this paper). Others have worked directly on ways to convert the unstructured data found in LLMs into knowledge graphs. (Here is a video about that.) Rao Kambhampati and his group at Arizona State have done some interesting work in trying to extract structured world models from LLMs to support model-based task planning.
6. For some, the truth of Christianity is "common sense," while for others it's not. Does this suggest that "common sense" is very context/culturally dependent?
As I mentioned in the talk, over the years (centuries, really) people have opined about what common sense is about, and no doubt different groups and cultures have different views on what it is. We have tried to distill the key elements (obviousness, shared knowledge, based on knowledge, practical, etc.) that we think are relevant to allow future AI systems to be successful in the real world the way that humans are when they appear to use common sense in everyday situations. But I don’t think there is any single set of beliefs/knowledge that we would call the definitive body of common sense. Things that appear commonsensical to one population and are part of the shared culture can be different from those to other cultures. I suspect there is a core that most if not all people would agree constitutes a shared common sense understanding, and I think that is the core of what the Cyc project has been about, for example. But it does appear to be the case that the full nature of common sense is different in detail in different places. This can even happen in expert knowledge domains. For example, I would suspect that once they are trained and deeply experienced in their own domain of expertise, NASA engineers would have a shared appreciation for things that appear to them to be commonsensical in their domain that untrained lay people would not know and would never consider second nature.
Find the next round of questions and answers with Ron Brachman here. Watch his full talk here or read a recap here.