Accelerating Drug Discovery with AI and Network Science: Recap
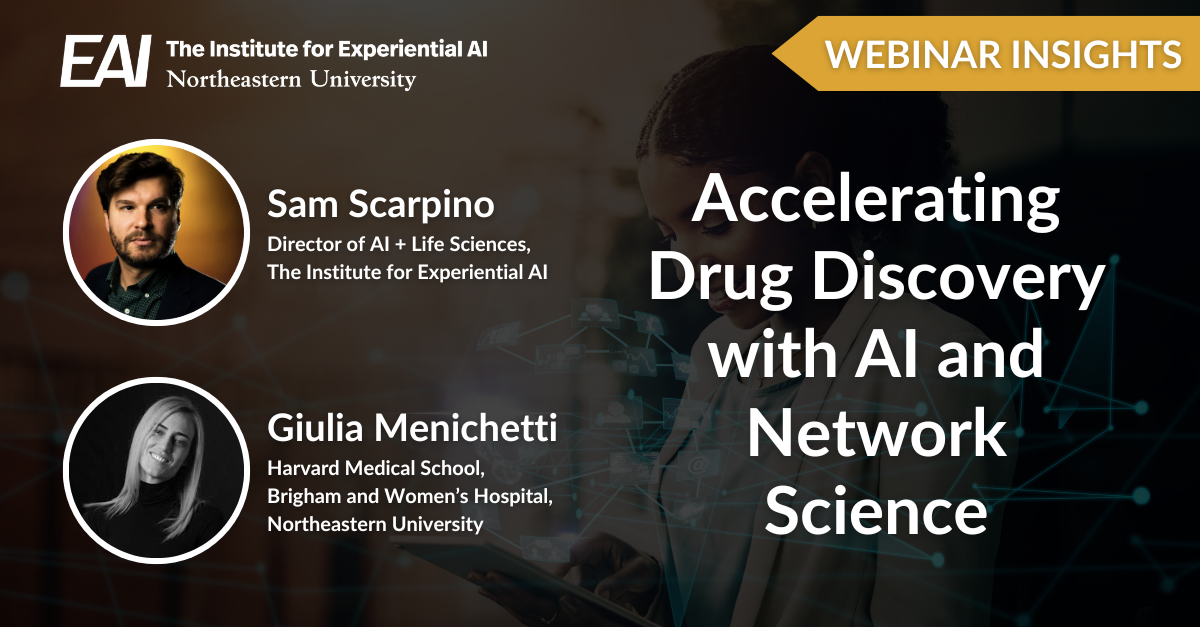
Nothing is independent from anything else. To understand how drugs interact with the human body, you need to understand a lot more than just the biochemical processes involved. You need to understand the complex dance of cellular, genomic, proteomic, and environmental factors at play.
That sounds complicated, but for Sam Scarpino and Giulia Menichetti, speaking in a webinar on the intersection of AI and drug discovery, unpacking the complexity of living systems is a cornerstone of network science.
As Director of AI + Life Sciences at the Institute for Experiential AI, Scarpino and his team use AI to uncover the nonlinear relationships that exist between discrete biological data sets — things like the genome, transcriptome, proteome, or phenotype. Life scientists call these “multi-omic data.”
Menichetti is a faculty member at Harvard Medical School, as well as an affiliated faculty member of the Network Science Institute at Northeastern University. As supervisor on the Foodome Project, she applies network science to unveil and map the mechanistic relationships between food molecules and human health. Characterizing the bioactivity of food molecules relies on tools compatible with drug discovery, and any methodological steps forward could inspire drug design and novel therapeutics.
Every scientific discipline deals with data, but the life sciences are unique in that they matured alongside the big data revolution. Machine learning, in particular, has been instrumental in scaling analysis of biological systems to the size of modern data sets. The problem is that, despite being large and complex, modern multi-omic data are still sparse. Consider that if each of our roughly 22,000 genes had only two possible mutations, that would still lead to over 200 million combinations. Now imagine that those genes could be turned on or off (gene expression) and the function of their protein products modulated by the environment. While we may have lots of data about these interactions, they are not sufficiently dense to accurately model living systems.
As Scarpino and Menichetti discussed in their webinar, a key to solving this data training challenge is having the right interdisciplinary team of human researchers “in the loop” and a different approach to training AI.
Complex Generalizations
In the webinar, Menichetti and Scarpino shared their perspectives on how AI and network science techniques apply to drug discovery. Of key importance was the finding that AI can be more effectively trained on sparse data coming from biological systems by thoughtfully building training sets using methods from network science.
For her part, Menichetti discussed findings from a recent paper in Nature Communications, where she and colleagues looked at how to model the effect of food molecules on human and microbial health. A necessary step was to characterize their protein binding partners, but unfortunately, the binding profile of food molecules is generally poorly annotated compared to standard drugs. Therefore, they needed to systematically predict new target interactions and identify a model to do it reliably and efficiently.
But AI models perform rather poorly when trying to predict whether or not a never-before-seen ligand—a small molecule that joins to a larger molecule to form a certain biological function—will bind to a protein target. In the words of the Nature paper, “state-of-the-art models fail to generalize to novel structures.”
What Menichetti’s research showed is that a more thoughtful, network-science approach to training and annotation can yield predictive insights that do not overgeneralize. What Menichetti’s team did was to leverage the network properties of the ligand-protein network collected for training purposes and generate more effective negative examples of binding. This strategy balances the types of annotations seen by the models and provides for each molecule at least one example of positive and negative binding. In other words, they defined a new strategy of data augmentation based on network science.
“We’re making sure that there is no huge bias in the overall type of annotations that the training displays,” she said. “In this way, step by step, you are shifting the relevance of what is learned towards the chemical features rather than the overall number of annotations, making sure that the model learns binding from first principles and consequently becomes better at generalizing.”
And it’s the magic of interdisciplinary teams—the “humans in the loop”—that distinguishes this kind of research.
The Curse of Living Systems
Studying living systems is in some ways a cursed undertaking, because you’re always going to be dealing with limited data. That’s partly because taking measurements is so expensive and complicated, but it’s also because biological systems are so small—there’s only so many observations that can be made.
One reason why Menichetti’s research is so exciting is because it shows how instrumental human expertise can be when inserted into the AI training process.
“If you're smart about how you build the training data,” Scarpino said, “the AI system will actually learn something that's more mechanistically relevant.”
And that, in turn, allows the model to create better, more accurate generalizations from highly complex sets of data.
The Institute for Experiential AI partners with laboratories, health care providers, pharmaceutical companies, and others to build AI solutions that reduce cost curves, improve diagnostics, and find new paths for drug discovery. Find out how we can collaborate on AI + Life Sciences projects here. Read Sam and Giulia's answers to audience questions here.