Closing the Gap Between Lab and Clinic: AI + Healthcare
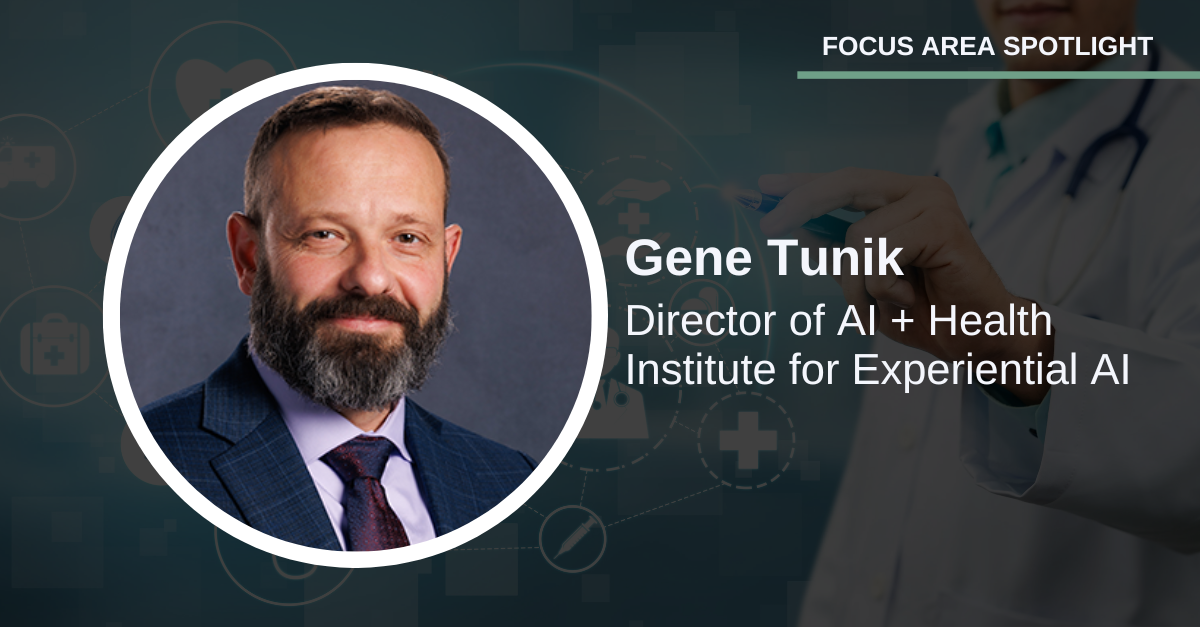
By: Tyler Wells Lynch
It may come as a surprise to learn AI is already used extensively in healthcare—not only in the day-to-day administrative work of hospitals and insurance providers, but in diagnostics as well. It makes sense. AI excels at finding patterns in massive datasets that are too large for humans to understand. When it comes to early disease detection, medical imaging, patient record analysis, and cutting-edge research, that kind of power is not to be overlooked.
But even as hospitals and labs across the world continue to implement AI algorithms to extend life and improve patient care, there are concerns about their reach. AI comes with myriad risks to patients and providers alike, and those risks cannot be ameliorated with more data or more robust algorithms. They require human intervention.
Eugene Tunik is the Director of AI + Health at the Institute for Experiential AI and the associate dean of research and innovation at the Bouvé College of Health Sciences at Northeastern University. His career-long interest in motor control, human-robot interactions, and neurorehabilitation led him, naturally, to AI, which he saw as holding enormous potential for his research focus.
“I saw a big gap between what was done in the research lab and what was practiced in the clinic,” Gene says. “The two were not informing each other in ways that were possible for truly great impact. I think that advances in digital health technology and AI have great potential to bridge this divide, transforming both research and healthcare.”
The Three Avenues for AI in Health
Gene’s interests reflect what he sees as one of three core promise areas for AI, the first and perhaps most obvious being research and diagnostics. Labs, hospitals, and universities can leverage AI to, for example, scan medical images for tumors that would otherwise be difficult to detect. From drug-discovery to 3D protein modeling to robot-assisted surgery, the research potential is enormous.
Beyond research, organizations can also use AI to assess, collate, and organize medical data. For an industry steeped in record-keeping, much of which still exists on paper, AI-assisted document review can be an invaluable tool. Similarly, the predictive analysis of machine learning models is vital to insurance providers, for whom risk assessment is the stock-in-trade.
But there’s a third potential for AI in the health space that Gene sees as wholly undeveloped, and it has to do with decision-making.
“I think the missing piece is the broad application of AI algorithms for large-scale clinical decision support services for the healthcare team,” he says. “It’s easier said than done, but that’s where the biggest need is—as a support system for healthcare providers to make decisions with their patients about how to care for an individual.”
Alleviating the Burden
Health is only becoming more and more complicated. Healthcare coverage is uneven and incomplete, as rural areas struggle with low patient-to-doctor ratios. Markets are often too dependent on primary care physicians for tasks nurses or assistants can manage on their own. Practices limit patient numbers to match profit objectives. And doctors are often preoccupied with menial work like clinical documentation, electronic record inputs, and regulatory compliance.
The potential of AI, Gene says, is not only that it can take over some of these routine duties but actually join the decision-making team and expand healthcare access.
“AI provides an opportunity to make healthcare much more accessible,” he explains. “Over the years clinicians have become so busy and the information has become so thick that they're just inundated, and so the patients are lost in the shuffle. Bad things happen when they're not attending to their patients, but I think AI has the promise of allowing clinicians to do the actual job they're trained to do.”
Do No Harm
As game-changing as AI may be, it is fundamentally a predictive system that derives its outputs from its inputs. That means any bias present in the cleaning or collection process will almost certainly be present in the system’s outputs. There’s a pithy saying about data and AI: Garbage in, garbage out. When it comes to healthcare, AI is no different.
There’s also the challenge of getting the public onboard. A recent Pew survey found 60% of American adults are uncomfortable with the idea of their own healthcare provider using AI to diagnose diseases or recommend treatments. It’s certainly not reassuring that AI has virtually no ethics infrastructure to speak of. In healthcare, physicians take the hippocratic oath. The National Institutes of Health restricts funding to certain types of research. New drugs need FDA approval before going to market. Medical researchers organize voluntary research bans on controversial topics like gene editing or gain-of-function studies. Nothing like that exists in AI.
“When you look at research and ethics oversight for human subject or animal research, those are well developed systems,” Gene says. “They’re imperfect, but we kind of know the imperfections reasonably well by now. I think that there's a lot to learn from these systems that can be adapted to oversight for ethical practices in AI.”
As Gene says, health is getting more complicated. New devices like wearables and sensors can monitor personal health with increasing fidelity. Lifestyle habits and diets shift with the culture. And new drug discoveries are being made all the time, sometimes with the help of AI. But the throughline here is data: it just keeps growing. And that, for Gene, is the source of his excitement.
“AI has the promise of allowing clinicians to look at aggregated data more comprehensively and more quickly,” he says. “It’s speeding up data synthesis to make clinical decisions, becoming a clinical decision support tool. That's where I think AI's greatest promises are.”
Watch a recent webinar with Gene discussing key developments in AI and sensing technology. Learn more about his research here.