Precision Oncology: Using AI to Find the Needle in a Haystack
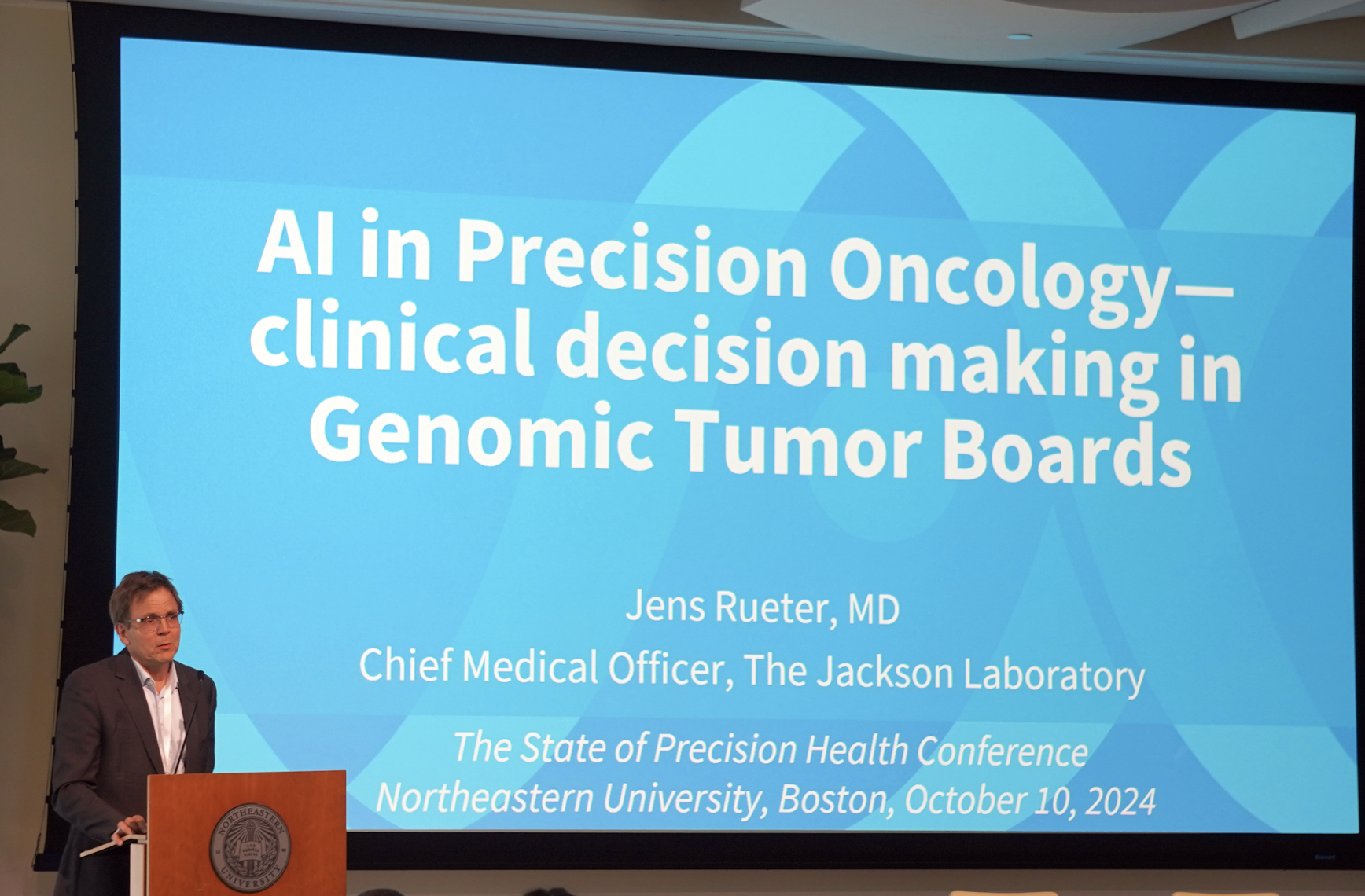
As chief medical officer at The Jackson Laboratory and medical director of the Maine Cancer Genomics Initiative, Dr. Jens Rueter has seen firsthand how important data is for improving cancer treatments and outcomes. He has also seen how overwhelming that data can be. Is artificial intelligence the solution?
In a talk at The State of AI in Precision Health on Oct 10, and in a later interview, Rueter explored how AI can improve clinical decision-making and even make healthcare more personalized—you just need to make sense of the data.
.png)
Too Much Data
Precision oncology involves the use of biomarker analysis—specifically at the level of the DNA, RNA, and proteins—to personalize cancer treatment plans based on the patient's tumor. While this kind of testing has become a standard of care, Rueter explained, it often results in complex and hard-to-interpret data. Clinicians are faced with lists of potential treatment options that may be overwhelming and difficult to prioritize. Such an "alphabet soup" of information makes it challenging for oncologists to select the best course of action for their patients.
“Finding the right treatment is a little bit like finding a needle in a haystack,” Rueter said. “So how can we help clinicians in making better decisions, and how can we help them find that needle?”
One approach The Jackson Laboratory took was to create genomic tumor boards (GTBs), which bring together experts in oncology and genomics to discuss complex cases and help guide treatment decisions. These boards review genomic test results and provide clinicians with treatment recommendations based on the most relevant scientific evidence.
Initially, the program was run in person, but it has since transitioned to virtual platforms, allowing for greater accessibility. The program has shown positive results, particularly in rural areas where access to advanced genomic testing and expert consultation is limited. The initiative has also demonstrated that patients receiving targeted therapies based on genomic tests have better health outcomes.
AI for “Boring Work”
However, the process is very labor-intensive, with a lot of manual work to organize meetings, interpret test results, and generate treatment recommendations. AI could help scale the process, assisting in managing genomic data, improving document intake, and providing real-time access to relevant publications and clinical trials.
“We can also provide AI assistance to the expert,” he added, “primarily in the beginning to refine this expert decision-making process. This whole concept of the expert in the loop, or the human in the loop, is something we can really facilitate here.”
While there are many challenges, such as the need for a standardized knowledge pool and greater trustworthiness of AI recommendations, Rueter has seen early promise in scaling genomic tumor board services and helping oncologists make better use of all that data.
“Oncology is a rapidly evolving field with new treatment options and new data becoming available on a monthly basis,” Rueter added. “At the same time, [oncologists] are getting more information than they know what to do with. So being able to have something to explain to them what might be the best option and why is going to be the most impactful system.”
Watch Jens’ full talk below or an interview with him here, and explore more coverage from The State of AI in Precision Health!