Seeking Out Problems For Maximal Impact
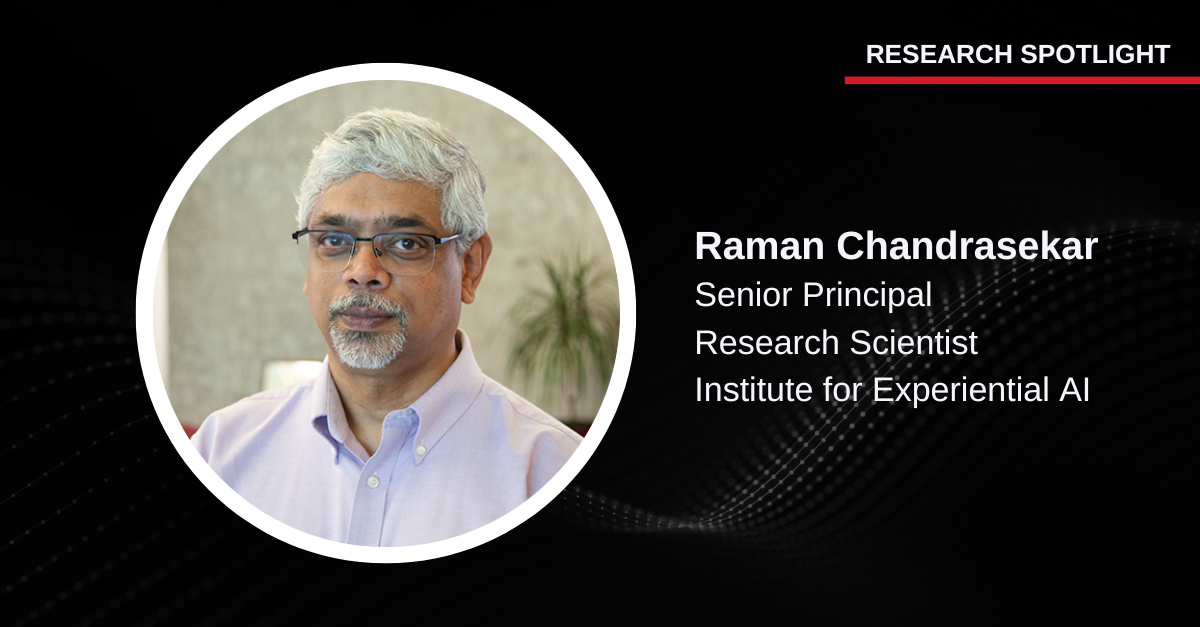
For years, Raman “Chandra” Chandrasekar kept a note on his desk that asked, “What is the problem I’m solving?” These days the note is gone, but the message still drives him. It’s what led Chandrasekar, a senior principal research scientist at the Institute for Experiential AI, to machine learning through the many twists and turns of his career.
“When I taught an artificial intelligence course at Khoury College, I always started with the Seeing AI example, where AI helps people who are blind or people with low vision use audio to visualize, experience, and engage with the world around them,” Chandrasekar says. “That’s my goal with AI: to look at concrete problems and solve them to make people’s lives better. That’s the reason for our existence.”
The technology solutions Chandrasekar uses to solve problems fall broadly into the areas of natural language processing, information retrieval, and machine learning. Since joining the Institute, he’s been collaborating with experts across all three fields on a wide range of projects that have piqued his interest.
“I loved the Car Talk call-in radio program that used to air on NPR,” Chandrasekar says. “The hosts were very educated, they fixed people’s cars, they helped people using their knowledge — and they had fun doing it! That’s what I want to do. I know some stuff, I want to help people with what I know, and I want to have fun doing it.”
Research With a Purpose
Before joining the Institute, Chandrasekar worked at Microsoft for 12 years, where he primarily worked on problems related to search and language, researching things like domain-specific search, news search, and improving systems for finding information on the internet.
Following Microsoft, he worked at Evri, a news dissemination startup, and in management roles at ProQuest, before joining the faculty at Khoury College, where he taught courses on AI, information retrieval, and deep learning. Through each position, Chandrasekar continued exploring ways of using AI to address problems and help people.
“The idea of working on AI and showing its capabilities is to me very important,” Chandrasekar says. “I make things and I want to make a difference in people’s lives.”
One area that’s captured Chandrasekar’s attention since joining the Institute is the world of academic publishing. While it’s rarely thought about outside of academia, the stakes are incredibly high for researchers. Decisions like who to hire, promote, and give tenure to are often based on metrics measuring their impact on a particular field.
But there are several problems with those metrics. The most popular ones use simple equations based on the number of papers published and the number of times those papers are cited. They offer a limited view of actual impact, and in part because of how impact is measured, sometimes quantity is emphasized over quality. In addition, these metrics don’t tend to account for changing citation practices, like the growing number of authors and citations used in research papers today.
People have also found innumerable ways to game the system by doing things like citing their own papers, listing thousands of authors, and paying to get their papers published. In fact, an entire industry of for-profit publication has sprouted up that accepts payment for publication.
“The current system is affecting the quality of research papers,” Chandrasekar says. “I think by developing more appropriate metrics, we can make academic publishing better.”
Chandrasekar is working to build better metrics with Institute Director of Research Ricardo Baeza-Yates, learning from and validating work using a large corpus of bibliographic data.
“Working on metrics is not sexy, but it can significantly impact publication and scientific discourse. We have to be principled and careful, keeping in mind that people rely on these systems to get promotions and tenure,” he explains.
Translating Tables
Machines aren’t (yet) very good at interpreting data from complex tables and charts, which is a problem for scientific, medical, and pharmaceutical research, where such tables are common. Chandrasekar sees an opportunity to create better programs through comparison.
“The problems are detecting tables in text, mining the structure of the tables, and finding the functional meaning of each cell,” Chandrasekar explains. “If they are complex tables, how do you understand that information and answer questions about it?”
If it’s a simple table, it’s relatively easy to for models to interpret each cell. For example, in a table showing information about various continents, the cell at the intersection of the row for Asia, and a column with the heading Population should provide the Population of Asia. But what if the heading spans multiple columns, or if a label spans multiple rows? What if some cell itself contains another table? Chandrasekar suggests we can learn from a large ‘parallel’ collection of papers, with each paper available in two formats: the academic LaTeX formatting which has a lot of structural information (identifying row and column labels, for instance), and the more common PDF format which includes page layout information.
To describe his approach to the problem, Chandrasekar tells the story of the time he was dating his now wife. Chandrasekar would go with her to the temple she attended, where the prayers were written out in Hebrew, transliterated Hebrew, and English. Chandrasekar would look at the transliterated Hebrew and English versions side by side, comparing the texts to try to learn Hebrew.
“That’s exactly what we’re trying to do here with machines,” Chandrasekar explains. “We’re looking at LaTeX [formatting] files and PDFs from a large parallel collection of scientific papers, and seeing what matches and how we can learn from that.”
By feeding models tens of thousands of LaTeX files, which define the formatting of tables, and their corresponding PDFs, Chandrasekar hopes to build machine learning systems that understand tables better.
“This is very much work in progress, and I believe it will take some time to get it right,” he says.
Broadening Horizons
Chandrasekar joined the Institute because it aligned with his thinking about AI. In addition to working with businesses to solve real problems, the Institute promotes a human-centric approach to building AI solutions, something Chandrasekar feels strongly about.
“As an institute, we believe in the human in the loop, and I firmly believe in that philosophy,” Chandrasekar says. “I really believe humans can do better than machines at many things.”
A focus on people changes how technology is designed and implemented — a theme Chandrasekar says defines his career.
“In a lot of the projects I’ve worked on, I’ve found the need for not just engineering but also good human interfaces,” he explains. “I think you can have the smartest idea in the world, but unless you craft it for the user, you’re not going to get much traction.”
More recently, Chandrasekar has studied the responsible use of AI, co-authoring a paper with Institute colleagues on the biases and addictive tendencies embedded in social media.
Working on the broader societal implications of technology is the latest pivot in Chandrasekar’s career. It’s an area that was made more personal for him with the recent birth of a grandchild.
That’s the kind of motivation Chandrasekar doesn’t need a note for.
Learn more about Raman Chandrasekar’s work here.