World Suicide Prevention Day: Can AI Help Researchers Better Understand Factors that Contribute to Suicide?
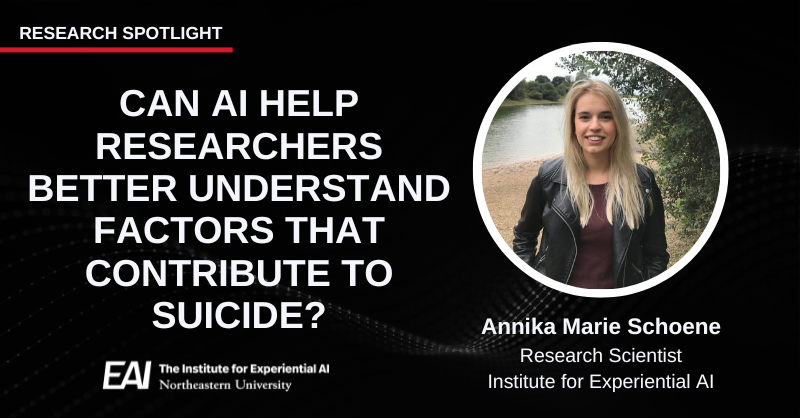
Studying mental illness poses a uniquely thorny challenge. Researchers have to rely on subjective reports and behavioral analysis to make diagnoses, but the idiosyncrasies of mental illness all but guarantee that the picture remains fuzzy. For suicide, the problem is even stickier, shrouded in privacy and unpredictability and regulated by a complex mix of social and individual circumstances that rarely point to a single, identifiable cause. Nevertheless, suicide rates are on the rise.
In the United States, where a majority of people lack access to mental health care services, suicide rates have been rising steadily in recent decades, reaching an all-time high in 2022. Some might squirm at the idea of using artificial intelligence (AI) to better understand or even intervene on suicidal behavior, but the severity of the crisis suggests all options are on the table.
Annika Marie Schoene, a research scientist at the Institute for Experiential AI, understands well the delicate nature of this conversation. Drawing data from public sources, she uses AI with a focus on natural language processing (NLP) to identify the signatures of suicidal intent and ideation on social media, helping her put a finger on the pulse of a crisis that shows few signs of abating.
In honor of World Suicide Prevention Day, we decided to sit down with Annika to learn more about her research and what it means for the mental health crisis.
Tell me about your work on NLP for mental health and how it relates to suicide. What makes this such a thorny issue?
Of course there is a connection between mental health and suicide, but a mental health diagnosis is not a necessary precursor to dying by suicide. More often than not, there are adverse life events or social determinants that can have a greater impact on someone dying by suicide compared to an existing diagnosis for example. My work mostly focuses on extracting information from mostly textual data that can tell us more about the kind of social determinants that could be contributing factors to adverse health outcomes.
If a person goes through a series of difficult life events—maybe they lost their job, have an unhappy marriage, had an unhappy childhood—these are all risk factors that increase the likelihood of someone dying by suicide. On the flip side, there are protective factors. If someone has a great social support network, lots of friends to take care of them, a financial nest egg—all of that reduces the risk. What I’m trying to do with NLP is to understand how people express this through language.
This kind of work is often quite challenging not just from a technical perspective, but also from an ethical and societal point of view. There is still a lot of stigma attached to suicide in any society and culture worldwide and in our discipline (AI) we are still in the process of putting guardrails in place for ethical processes, standards, and regulations.
Do you see any real-world application areas for this kind of research?
There are some clinical application areas, but also less well known ones. For example medical professionals may take notes in electronic health records (EHRs) to record if someone struggles in their job or financial well-being. Officially, there are codes for this in the ICD-10 called Z codes, but recent work has shown that less than 2% are actually utilized, which is mind-blowing, given all this massive amount of research that purports to identify risk factors. One way we could use NLP is to automatically extract this information and populate EHRs to help clinicians see the most important information upfront, which can help them with identifying risk or diagnosis.
What kind of data sources can you use?
Research shows that many people don't actually express their mental health issues or suicidal ideation to professionals even when asked. So if you're a mental health professional or a clinician and you ask your patient directly, “Do you have thoughts about suicide?” Many say no when the reality might be very different. What people tend to do is go on social media and describe their lived experience. They may post online about a horrible job or a bad breakup. With NLP, what we can try to do is develop domain-specific named entity recognition models that automatically extract that information in a de-identified form to learn what factors influence adverse health outcomes.
Another application area would be to use publicly available data to take the temperature of the public when it comes to mental health. During the COVID pandemic a lot of research came out suggesting the mental health crisis is getting worse, and suicide is just one element of it. Mental health struggles are obviously far and wide, and suicidal thoughts itself can co-occur as a symptom with other diagnoses.
However, this is a very fine ethical line to walk. Data, language models, and other available resources often are not properly validated or used in the right context. This casts doubt on how much value using AI in this setting can add.
There seems to be a tension at the core of this work between the right to privacy and the use of information to illuminate trends in mental health or suicide. How do you navigate that?
Yes, that tension does exist. Take my work as an example: I try my best to adhere to standards of data regulations and guidelines. Some measures that I am taking include not storing usernames or profile information. I mostly care about the actual free structured text and try to code stuff to ensure that references to, for example, other users in a tweet are not included. I also don’t tend to make my resources publicly available and only share data upon request (if appropriate and for non-commercial use) and within the legal framework of each platform. However, user privacy is only one aspect in which there are ethical tensions in NLP for mental health research.
There’s also ethical tension in how this type of data is made publicly available and if it contains any ground truth. If you go on Kaggle (a popular website for data science competitions) right now and you search for available mental health datasets—you don't even have to put in suicide—you will find plenty of language and tabular datasets that contain references to mental health conditions like depression or suicide.
Initially, you may not think that this is a big deal, but in reality there are a number of questions that arise from this, such as: Is this a dataset verified by medical professionals? Or did someone just randomly assign labels of conditions or risk to a piece of text? And if this dataset is not verified or evaluated by professionals, how are we training language models to learn? And what happens if we use these pretrained language models in a more serious setting?
We can go on Huggingface (a company that provides open-source machine learning technologie, such as large language models and datasets) and find quite a few pre-trained language models that have been trained on various types of mental health related data, and we often don’t get to see the model cards for it. (Model cards are a means of reporting how a particular model has been developed, including contexts and situations in which it would be unsuitable.) There are many unintended consequences of using this data and I personally don't have all the answers to solve this.
These are all really important questions, where there's currently no real ethical guidance. I am collaborating with Institute members Cansu Canca and Laura Haaber Ihle about establishing ethical guidelines to conduct more responsible research in this area.
Learn more about Annika here.