AI in Health and Life Sciences: Challenges and Opportunities
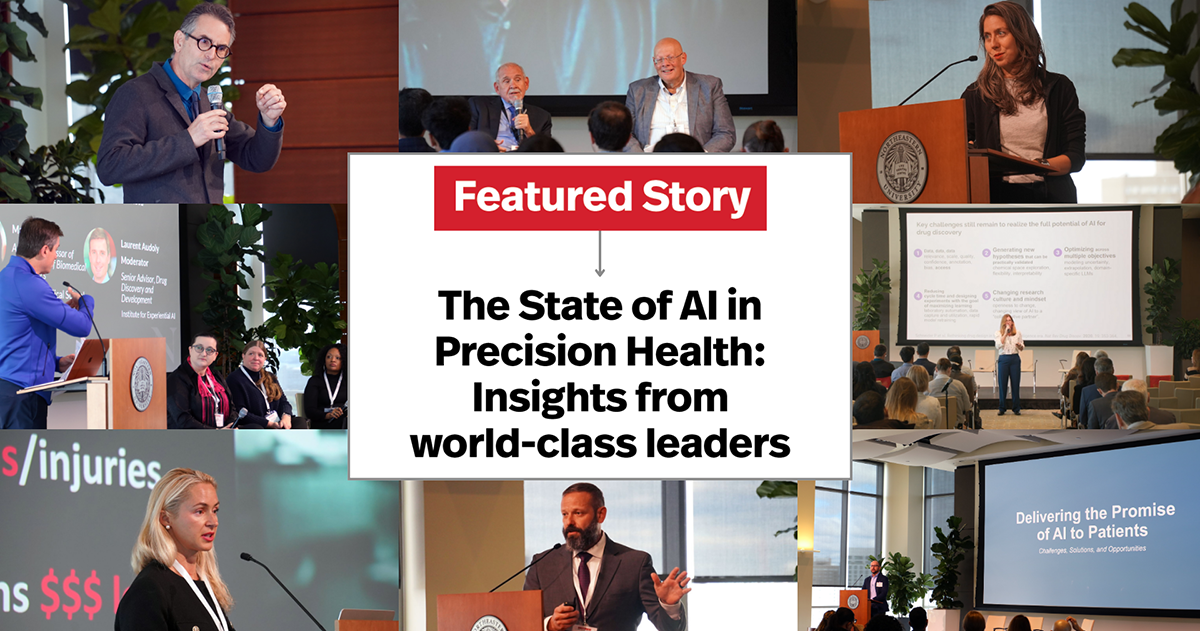
This month, we hosted world-class organizations including Advocate Health, Boston Children’s Hospital, Evity.AI, Harvard Medical School, Mass General Brigham, Mayo Clinic, The Jackson Laboratory, and more, at Northeastern University for The State of AI in Precision Health conference.
Industry leaders provided an incredible look into how they are leveraging AI to accelerate new drug discoveries, increase efficiencies, enhance healthcare delivery, and more. Key discussions addressed challenges, debunked AI hype, and mapped the future of AI-enabled health.
In this issue, we explore insights from The State of AI in Precision Health.
Longer lifespans are changing the nature of healthcare, but AI could help humanize care, cut costs, and provide more time with patients.

“While longevity has been increasing—it doubled over the last 100 years in the US—that longevity has not been paired with an increase in healthspan. Today, Americans are spending a higher proportion of their life in sickspan than ever in history… The demographic transition is even more important: a world in which there are many deaths, many births, and a high growth rate to a world in which there are few deaths, few births, and a slower growth rate."
"‘In the loop’ means creating decision support systems that help clinicians make better decisions that help physical therapists make better decisions that help you make better decisions.”
- Larry Brilliant, CEO, Evity.AI
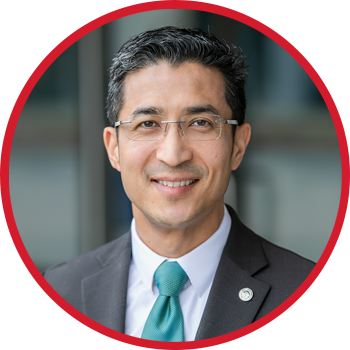
“Artificial intelligence has the power to humanize care… At our medical school, we're bringing design thinking, empathy-based learning, health equity elements, and AI into the curriculum. We're bringing in IRCARD North America, an experiential learning surgical simulation robotic and AI institute that'll be the focal point for a variety of different companies and systems to work together around robotics and minimally invasive surgery. The health ailments of tomorrow will not just be fought with x-rays and stethoscopes. They will in fact be cured with data and AI.”
- Rasu Shrestha, chief innovation and commercialization officer, Advocate Health

“AI represents one possible area where we can invest to reduce our costs, reduce the burden that we place on our providers, and up the patient experience. We already know what the value proposition is for healthcare and AI—the idea of improving productivity, reducing burnout, improving the patient experience through new tools. There's this view that clinical outcomes can be improved, because now we have a co-pilot sidekick alongside every clinician, but cost reduction is a big one: Where can we bring in the tools that will support the reduction of the massive amount of cost that it takes to take care of our patients? It’s about what we need to do to upskill an entire institution to take advantage of these tools and to reduce the cost that we are spending taking care of our patients.”
- John Brownstein, SVP and chief innovation officer, Boston Children’s Hospital
Drug discovery is time- and resource-intensive, but better data could allow for more sophisticated drug target identification

“The cost now of bringing a new drug to market, when you capitalize for all the failure, is over $2 billion. If we think about drug pricing and why things are so expensive and we look at the time it takes to develop new drugs — 10 to 15 years plus a patent life of about 20 to 25 years — that doesn't leave companies much time to recoup the money they've invested. And these numbers keep going up, so as we've brought technology to bear… we are still not doing better, and in fact we're kind of doing worse.”
- Melissa Landon, technology and business strategist, AI in Life Sciences
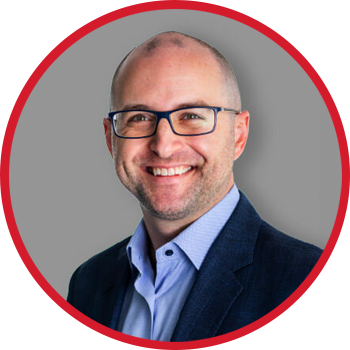
“We’re really getting to the cusp. In the next few years, we're going to start to see hundreds of products designed by AI get through those phase two trials. The challenge we're facing right now is we still don't have any better data. If you want to see the day that the ability to design new molecules—small or large—delivers higher impact in phase two and phase three trials, being able to better understand what targets and diseases you're actually treating I think is the key to making it better, because it will enable you to actually improve those phase two results.”
- Jason Springs, co-founder and CEO, Endpoint Health
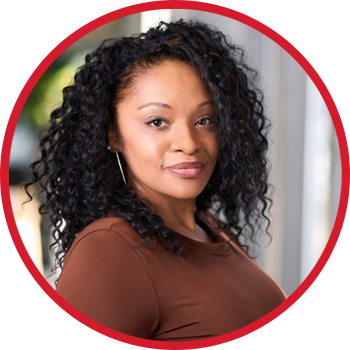
“One of the things that I'm most excited about at CircNova is that we're leveraging AI to reduce cycle times, flatten cost curves, and have new biological insights that allow us to not only design novel non-coding circular RNAs, but also predict what those structures should be. When we look at the amount of limited structural data that's been around for the last several years, what we're able to do now is on a whole different playing field. It allows us to be able to pursue therapies that did not exist before. What we're constantly focused on is making sure that we're looking at the right targets, so we can have success in delivery and success when it gets to preclinical trials.”
- Crystal Brown, co-founder and CEO, CircNova
At-home patient monitoring is only as good as the data available, but AI-assisted monitoring devices can produce more and better patient data.
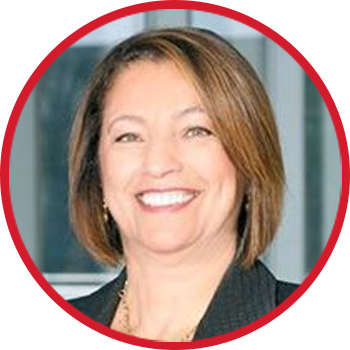
“If we think about the data being used to train LLMs, a lot of it is coming from medical records. What isn’t being used is data directly from the patient, [because it’s of low quality]… We have to look at patient-generated data more carefully. Wherever it comes from, we need to gather that data and train our AI models on this different class of at-home patient data, and there is a lot of it.”
- Hoda Sayed-Friel, former executive vice president, MEDITECH
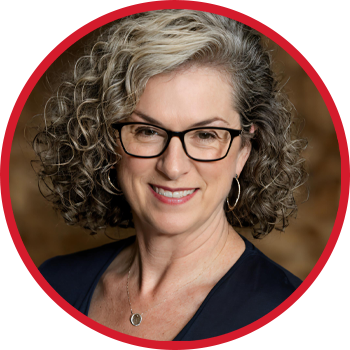
“It’s easy to monitor a patient in the ICU bed because they don’t move, but the nature of caring for patients in their home is different. They do move better and fall less frequently, they get fewer infections, less delirium or insomnia, they eat better, move better, sleep better. But now I don't have control over how they're moving. I want to monitor their fall risk, predict their fall risk, and I want to know when they fall, and I want to learn how to mitigate their risk. That's where I think there's a lot of opportunity — to create more sensitive monitoring devices and make meaning of all of that data. It doesn't really do me any good to get a bunch of data points if I'm not able to make meaning of it.”
- Colleen Hole, innovation strategic advisor, Advocate Health
AI has a long way to go before it’s part of the healthcare team, but explainability and interaction are key to its success in clinical settings.
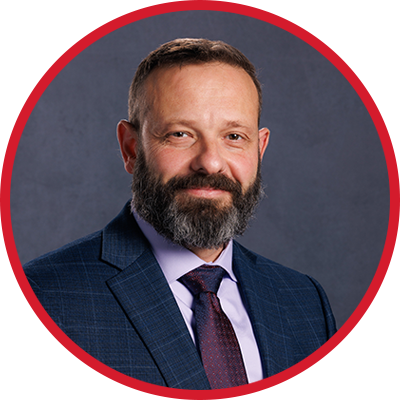
“What do we need to enable [the promise of AI in health]? We need to have the boring work of scheduling, documentation, billing, all of that kind of stuff figured out. We need to have real time-ish data curation with predictive models running in the background to help inform clinical decisions. We need to have a means by which to characterize disease and guide interventions… A key point here is going to be whether these technologies are going to be able to interface with humans the way a human would… And finally, we need to invest in educating the future clinical workforce in being able to talk to our computer scientists, data engineers, and so on, because otherwise the fields are going to go in parallel silo tracks.”
- Gene Tunik, director of AI + Health, Institute for Experiential AI
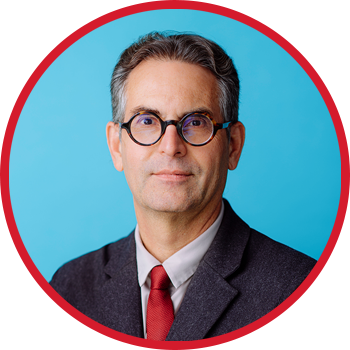
“How do we know a model works? How do we know if it's fair, appropriate, valid, effective, and safe? Well you need to test it, and mathematically you can test a lot of the predictive AI against different data sets… Every model will be biased and that's okay as long as you understand what the bias is and mitigate the bias.”
- John Halamka, Dwight and Dian Diercks president, Mayo Clinic Platform
AI has incredible potential in addressing complex health and life sciences industry challenges.
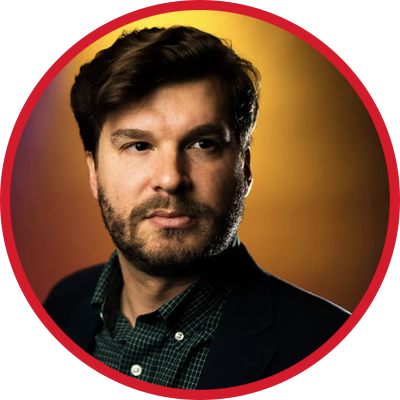
“The actual secret sauce is a lot of really good project management and tight coupling of wet lab and dry lab. That's what we mean in the life sciences by experiential AI: humans and wet labs in the loop. I think that because of the responsiveness and the historical contingency associated with living systems, the only way we can study them is by constantly measuring and remeasuring as we perturb the system through treatment, as we look at different populations of individuals that are carrying along with them decades, generations of historical contingency. That's what we're focused on in the institute for experiential—partnering with startups, pharmaceutical companies, governments, nonprofits to bring these kinds of expert, wet-lab-in-the-loop AI systems to bear on the world's largest, most pressing problems, and then identify opportunities to roll back research into the university.”
- Sam Scarpino, director of AI + Life Sciences, Institute for Experiential AI

“What remains to be done is to tackle the problem of when are [patients] going to have their adverse event? Can we build predictors that look at the next eight hours or four hours or whatever the time increment is and build a system that says, ‘That patient there is highly likely to undergo a specific adverse event in the following time interval.’ [With AI,] that is actionable.”
- Rai Winslow, director of life science and medical research, The Roux Institute
.png)