Recap: The Narrow Road for AI in Drug Discovery
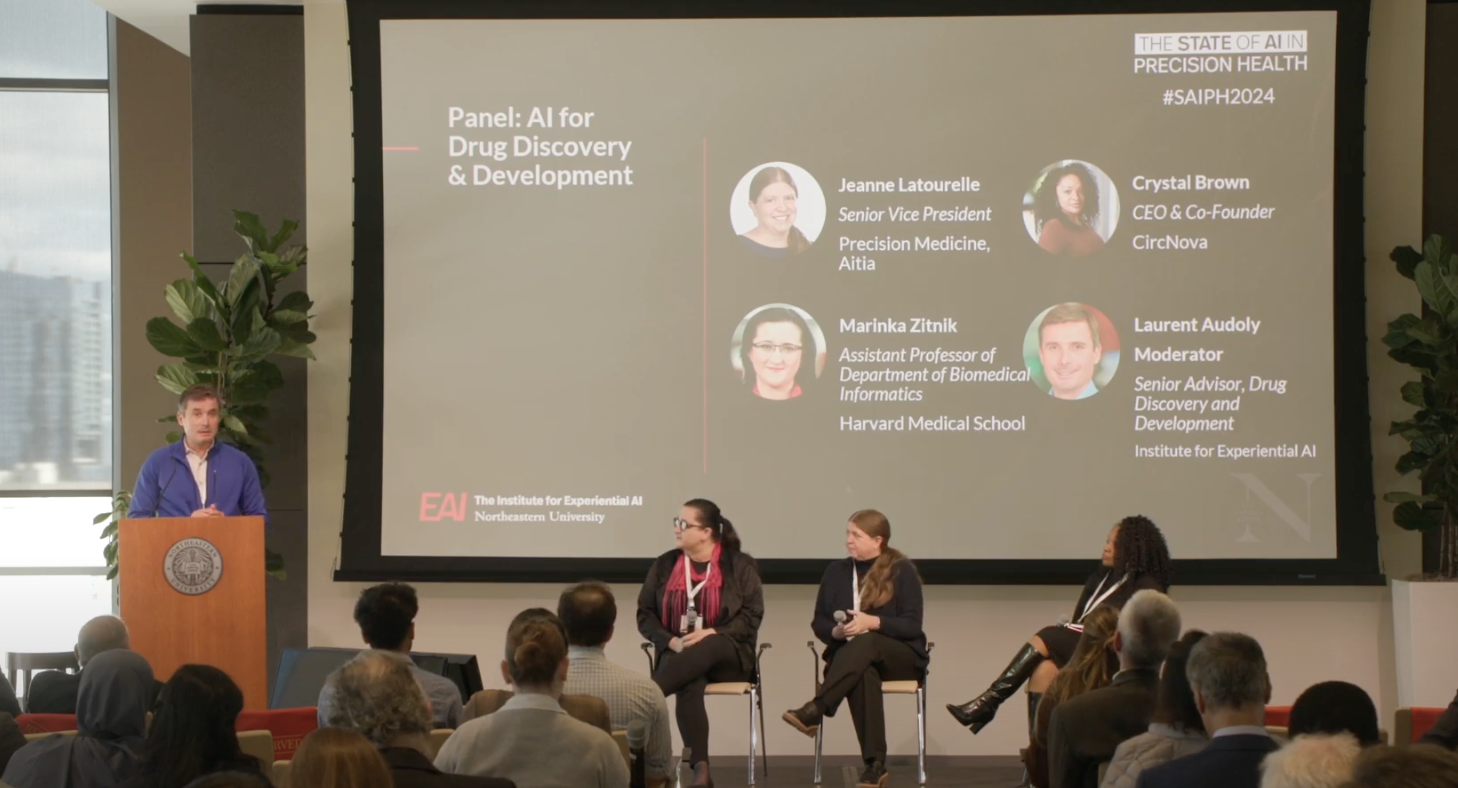
The road to market for a new drug is long, winding, and expensive. In the end, only about 2 percent of drugs make it, and the attrition rate for new compounds is only growing. While artificial intelligence has injected some much needed enthusiasm into the drug discovery space, skepticism remains.
Of particular concern is the basic fact that drug discovery seems to be getting more difficult. It’s taking longer and longer to bring new compounds to market, and it’s costing companies more money to get there—on average about 15 years and $1 billion. But it’s not all doom and gloom. In fact, artificial intelligence is well positioned to deliver the age-old dream of personalized medicine.
.png)
The State of AI in Drug Discovery
Speakers and panelists shared these thoughts and more at The State of AI in Precision Health, the marquee AI, health, and life science conference hosted by the Institute for Experiential AI on Oct. 10. The event included talks on drug discovery and personalized medicine from leading executives, biomedical professors, and other drug discovery experts.
Together, participants offered a blueprint for success in drug discovery, stressing the importance of well-curated data, cross-disciplinary collaboration, and a kind of “learning agility” among scientists, researchers, and stakeholders. They also stressed the need to be realistic.
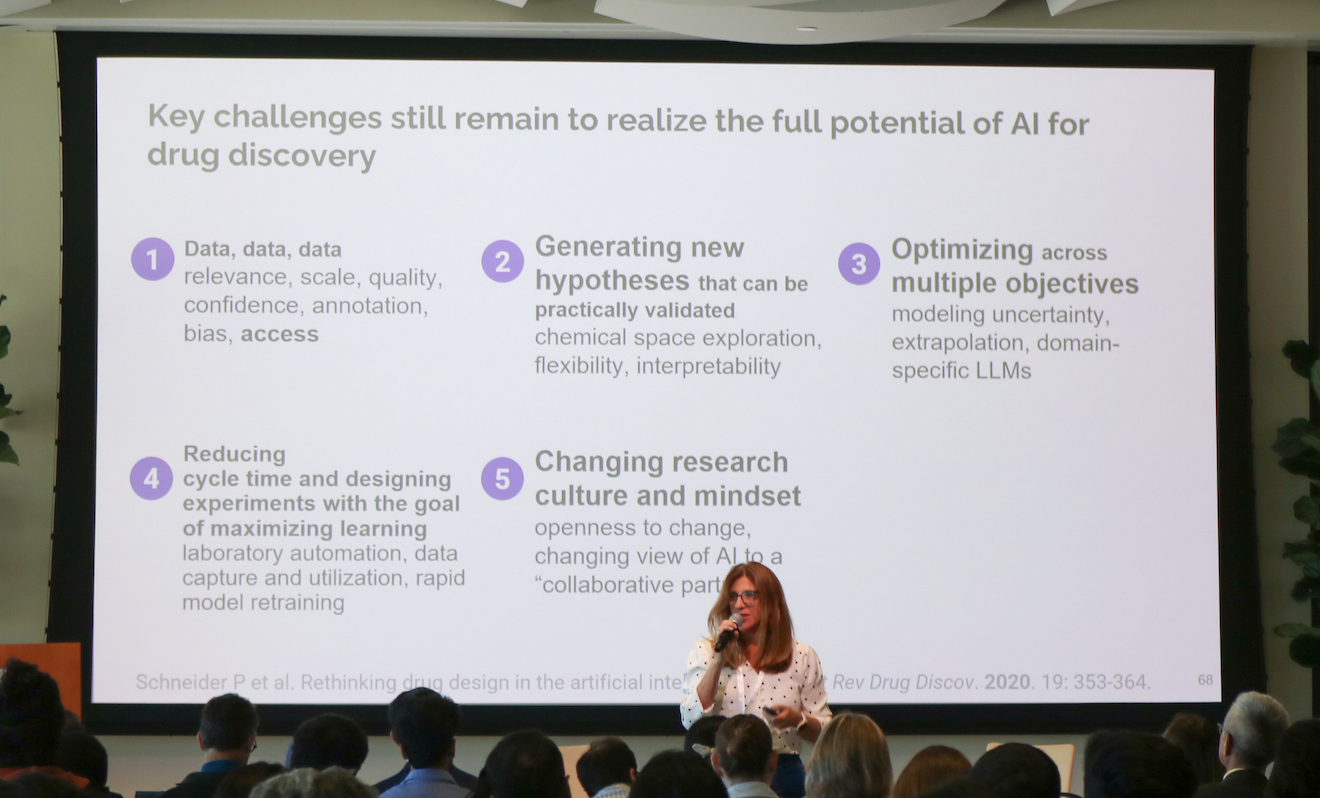
“The cost now of bringing a new drug to market, when you capitalize for all the failure, is over $2 billion,” said Melissa Landon, a technology and business strategist with a background in molecular biology and computational chemistry. “These numbers keep going up, so as we've brought technology to bear… we are still not doing better, and in fact we're kind of doing worse.”
It’s worth noting the idea of using machines to design drugs is not new. For instance, as Landon pointed out, a 1981 cover story in Fortune magazine was about the potential of computational methods in drug discovery. Although the algorithms we employ today are more sophisticated, the foundational ideas have been around for decades. Currently, we find ourselves in a "hype cycle," where the initial excitement surrounding AI in drug discovery is beginning to wane. The burden of proof is high, and while we are starting to see some impact, it has yet to meet expectations.
But, as skeptical as Landon is, she is far from a pessimist. She pointed out how the most recent Nobel Prize in chemistry went to three scientists using AI to invent new proteins and predict the shape of known ones, and the Nobel in physics went to discoveries in machine learning. Increasingly, AI is being used to reduce attrition rates through improved biomarker identification, optimized clinical trial design, and the development of fully synthetic control arms in trials.

Others at the conference were perhaps even more bullish. Jason Springs, co-founder and CEO of Endpoint Health, stressed the potential impact on patients, going so far as to predict that, in the next few years, we’ll start to see hundreds of products designed by AI getting through phase two clinical trials. We just need better data.
“If you want to see the day that the ability to design new molecules—small or large—delivers higher impact in phase two and phase three trials,” Springs said, “being able to better understand what targets and diseases you're actually treating I think is the key to making it better, because it will enable you to actually improve those phase two results.”
Behind the Scenes
AI in drug discovery isn’t all target identification and protein prediction. It can also help facilitate backend processes like document review, knowledge synthesis, and administrative routines. Jens Reuter, chief medical officer at The Jackson Laboratory and medical director at the Maine Cancer Genomics Initiative, imagined an AI interface for genomic tumor boards (GTBs):
“Can we build an AI interface that will enable real-time access to relevant publications and to open clinical trials that are actually enrolling, so that information can then be fed back into the GTB discussion in real time, and not require any additional manual searches or delays, and also provide a consistent source of information?”

Some of these ideas were explored further in a panel moderated by Laurent Audoly, senior advisor of AI drug discovery and development at the Institute for Experiential AI. It included Landon, as well as Crystal Brown, CEO and co-founder of CircNova, and Marinka Zitnik, professor of biomedical informatics at Harvard Medical School.
Brown’s background in automotive manufacturing gives her a unique perspective. She noted the importance of embracing failure as part of the learning process and emphasized the importance of selecting the right therapeutic targets early on in the drug discovery process.
“One of the biggest challenges when it comes to leveraging AI is there is the theoretical potential and then there's the implementation of what you can actually do,” Brown said. “If I had a blank slate and could do things differently, the truth is I would do nothing differently, because the failures are necessary for the successes.”
A Ginormous Multiparameter Optimization Problem
Marinka Zitnik spoke at length about some of the laboratory challenges of drug discovery and where AI can help bridge the gap between biological research and clinical outcomes. She emphasized the importance of understanding molecular functions within biological environments, noting that while predicting molecular structures with AI has been successful, understanding their functionality remains a challenge.
Another persistent challenge lies in data accessibility: As the drug discovery process advances, the data required becomes more expensive and less scalable. It doesn’t help that a lot of that data is locked away by big, protective pharmaceutical companies, unavailable to researchers who aren’t on the company dole.
As Landon put it, drug discovery is a “ginormous multiparameter optimization problem,” because a successful molecule has to make it past a gauntlet of biological, legal, environmental, and commercial obstacles. Currently, there is no drug on the market that has been designed by AI. But the success rate of AI-assisted new drugs in Phase 1 clinical trials—where researchers assess safety and, to a lesser extent, efficacy—has improved significantly. In Phase 2, the success rate still mirrors that of pre-AI levels, but the speakers highlighted some of the things that need to happen for broader impact.
One of them has to do with data. Harnessing the power of AI in drug discovery is going to require nuanced understanding and partnership across many disciplines. That means opening the research field to allow for more shared resources, data commons, and protein banks, as well as a collaborative ethos that bridges industry and academia. Feasibility, it seems, has as much to do with mindset as it does technology.
“I do think mindset has changed significantly over the last few years,” Landon said. “Most scientists I talk to are very happy to adopt and embrace AI and computational technologies if they're going to be impactful, but they need to be impactful.”
Learn more about how the Institute for Experiential AI is putting the human back in the loop of AI and drug discovery, and be sure to check out all of our coverage of The State of AI in Precision Health conference!