Using AI to Find the Link Between Traffic and Germs
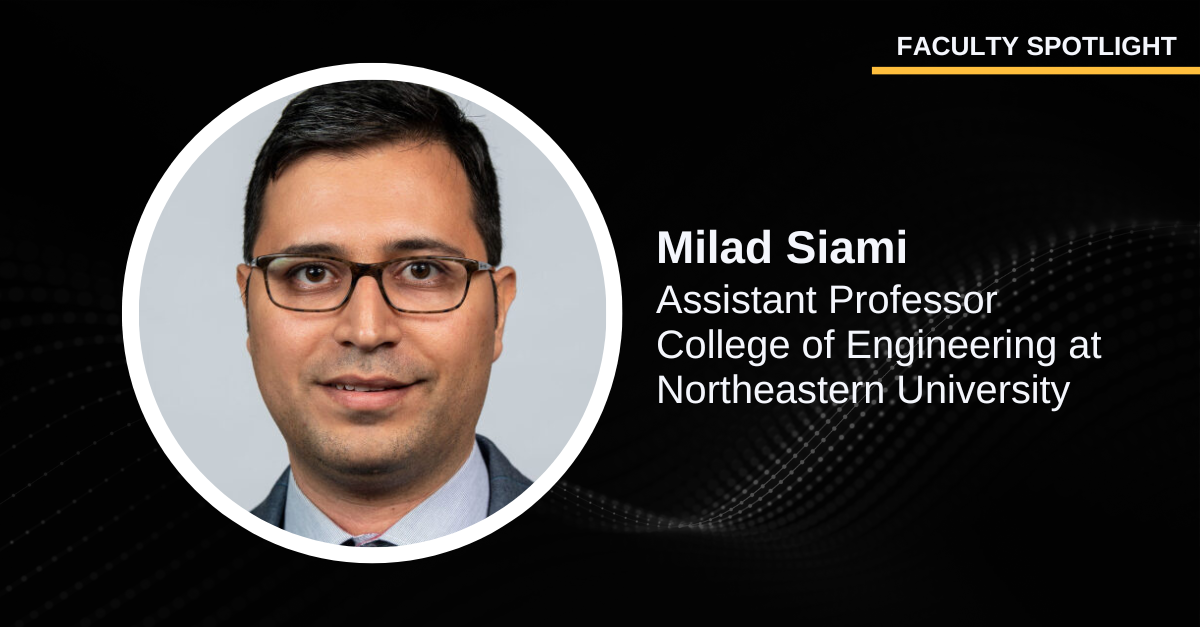
Data is fundamental. Sometimes called the “raw material” of the information age, it allows us to convert isolated inputs into highly accurate predictions about how the world works. Whether it’s a traffic network or a disease outbreak, the more complex the system, the more data is needed to model it—but not all data are created equal.
For Milad Siami, artificial intelligence is first and foremost a tool for uncovering these kinds of hidden states. As an assistant professor in the College of Engineering at Northeastern University, he’s interested in the underlying structures of large-scale networks. With the help of machine learning (ML), high-performance computing, and robotics, he and his colleagues work to make networked systems more reliable in highly uncertain environments.
“We're working on self-driving cars and robotics,” Siami says, “and we’re working on epidemic and pandemic prevention, but in all those applications the goal is to understand the behavior, make them robust and design them in a better way.”
The upshot is better predictions in chaotic settings. As a core faculty member at the Institute for Experiential AI, Milad champions AI approaches that center the human experience. Earlier this year, a robotics team he led from the electrical and computer engineering department won first place at the 2023 American Control Conference (ACC) Self-Driving Car Competition—one of the most prominent showcases for students working in controls, robotics, and machine learning.
While the contest was all about improving the automotive capacities of networked systems—in other words, making them work without manual control—the human element was never far from mind. After all, cars have to operate with and around people. But even in the most ideal settings performance is contingent upon human oversight and intervention.
“There are a lot of challenging problems in AI,” Milad explains. “They’re multidisciplinary problems, and they need different viewpoints. Even if the theoretical gist is the same, the application domains are totally different and we need expertise from each to implement results.”
For Milad, this prestigious award taught a critical lesson about the complementary relationship between knowledge diversity and system operability. Building more robust algorithms isn’t just about inserting manual oversight into the training process; it’s also about making systems more explainable to human operators. And that may be the greatest challenge of all. Whether it’s in a self-driving car or a model of a disease outbreak, “black box” algorithms can carry harmful biases, frustrate efforts to improve them, and compound the mystery of systems that AI is supposed to help reveal.
“We want to have some sort of explainability in our algorithms to make sure it works well,” Milad says. “And we want to make sure everything is ethical and fair. Those are very rich problems that need to be addressed.”
Despite his team’s win, the field of autonomous driving remains a dicey one. Headlines abound with concerns that the field has stalled. Last year, Ford and Volkswagen shuttered a joint effort to develop self-driving cars, while Google put a pause on its self-driving truck division. A report by F-Prime Capital, meanwhile, found investments in autonomous vehicles declined nearly 60 percent in 2022. What’s going on? Are we experiencing an AV winter?
Autonomous vehicles are very good at driving in controlled environments, but real-world traffic conditions are anything but controlled. They’re chaotic in ways human drivers take for granted, adapting on the fly to changing conditions on the road. AI has struggled mightily with that level of adaptability, and the challenge in the field is now about making computer vision and autonomous driving systems better at reacting to rare events.
“We need to make sure we can handle those rare events,” Milad says. “This is a very hot topic to re-tech and mitigate rare events in complex networks. It's very critical because people are part of these systems and we want to protect them.”
That research vision is part of Milad’s larger interest in finding the underlying patterns in data. It may not be that the best algorithm is the most efficient algorithm, but rather the one that is best able to respond to changing parameters or even adversarial interactions. That’s true for a high-traffic environment as much as disease outbreaks, where state changes are highly irregular and difficult to predict.
“We can experience failures in different components of an autonomous system,” Milad says. “What we want is to make sure everything still functions properly in the end. That means we aim to make it robust against changing environments or changing parameters or even adversarial attacks.”
In biology, they call that evolution.
Learn more about Milad’s research and how the Institute for Experiential AI is working to solve data problems.