On the Dangers of Stochastic Parrots: A Q&A with Emily M. Bender
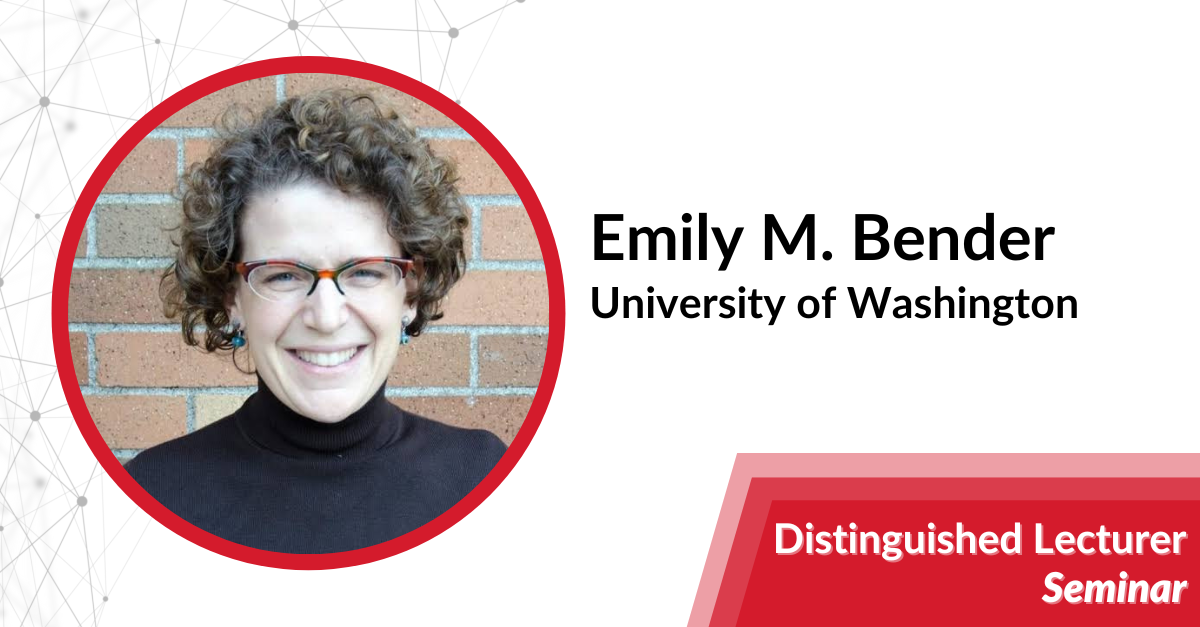
By Tyler Wells Lynch
The Institute for Experiential AI welcomed Emily M. Bender, the Howard and Frances Nostrand Endowed Professor of Linguistics at the University of Washington, to speak about the risks associated with language models in the field of natural language processing (NLP).
Language Models (LMs) are used in machine translation, speech recognition, information retrieval, and tagging. In the era of big data, Large Language Models (LLMs) represent a new frontier in artificial intelligence and machine learning. But at what cost? As part of the EAI’s Distinguished Lecture Series, Emily Bender revealed how large data sets marginalize “low-resource” languages, aggravate global CO2 emissions, and fortify machine biases, even when they’re meant to combat them. In turn, latent biases reinforce hegemonic viewpoints and raise important questions about the present course of NLP research.
After her lecture, Bender took time to answer questions about next steps for language modeling and what alternative research paths are available. To learn more, you can read a written recap of the lecture or watch the video replay.
Q: If the aim of being fair in language data is to have a real-world representation of all categories of gender, race, beliefs, and so on, how does one go about building such a data set, especially when a large part of the data does not come with this identifying information?
Emily M. Bender: There’s two ways I’d like to shift the question before answering it. The first is, there is no such thing as any dataset or model that’s completely unbiased. A lot of this is just about harm mitigation. But there are things that we know we can do better on, and we should do those things, but we should also never lead ourselves to believe that we’ve created something that’s fully unbiased.
The other part of the answer takes issue with the ideas of generality — what the folks at Stanford are calling “foundation models.” If we try to build something that is usable in all situations then we have set ourselves up with the impossible task of making something that would be fair in all of those situations. If, instead, we’re building technology for specific contacts in specific communities, then we can reason from: What’s going on in this community? What are the risks that we see here? How is this technology going to be used, and how do we build it so it can be used safely?
Q: Is there anything we can do to stop the use of large language models by bad actors?
EB: I certainly don’t have solutions but I have a few thoughts. My colleague Ryan Calo at the Tech Policy Lab here at UW has some wise things to say about how, when you’re regulating technology, it’s important to regulate affordances and not techniques, because the techniques are going to keep changing. If you want to make durable regulation it has to be about actions that people take with the technology or things that the technology allows them to do, and not the particular ways the technology does it. Again, channeling Ryan Calo, there’s questions of figuring out to what extent existing regulations already apply. Let’s not be overly impressed with the technology and assume we can’t possibly handle it with our existing regulations.
You can reach well-intentioned people doing harmful things by giving them things to think about so that their good intentions actually lead to good things. Bad actors with ill intentions raise the need for effective regulation. Individuals should be in conversation in terms of educating the public so that people know what’s going on. Then, when we get the opportunities to talk with policymakers the conversation is already there. It takes a lot of expertise to build effective policies, so I don’t pretend to know how to do that, but I believe that someone who knows how to make policy doesn’t necessarily know the domain stuff that I know. If I can collaborate with them we can make effective policies.
I also wanted to say that I remain upset with the way Google treated my co-authors on this paper. I think it’s really unfortunate in many ways, but a silver lining was it brought this paper to the attention of the public. I have been grateful for the opportunity to speak to the media and to let people know what’s going on. I think it’s important for there to be voices who are educated about this technology and pushing back against the AI hype. So much of what we read about language technology and other things that get called AI makes the technology sound magical. It makes it sound like it can do these impossible things, and that makes it that much easier for someone to sell a system that is supposedly objective but really just reproduces systems of oppression.
Q: What role can the creative arts play in exposing and mitigating potential kinds of creative language models?
EB: I want to point to the Algorithmic Justice League, which is led by Joy Buolamwini, who you may know from her famous workaround face detection and how it doesn’t work for dark-skinned people. She does amazing work using creative arts to bring various issues around bias and technology into the public consciousness. A fantastic role model in these lines.
On language models, in particular, there was recently some sort of a play that was written each time by one of the language models, and the actors were speaking the lines that were produced by the language model. And it was coming up with all kinds of offensive stuff, but from what I’ve read, it seems like it was framed pretty well — not to just shock people, but actually to expose and educate. But absolutely I think creative arts are really important in this space.
Q: Is there anything you can suggest in the way of a research program for scholars who care about the texts being used to train language models for their own sake? When we think about different directions that research could go in, how could these fields contribute in ways that go beyond reinscribing existing patterns?
EB: In the proposal that Friedman and I put together called “Data Statements for National Language Processing,” which is one of these documentation proposals, we are drawing on sociolinguistics and other subfields of linguistics to work out what aspects of the speakers, the annotators, and the speech situation should be documented to help people understand the potential sources: on the one hand, sources of bias; on the other hand, the degree to which something trained on that data set would generalize.
I think that sociolinguistics in particular is a very useful field for people working with language technology to be educated in and draw on. In the other direction, there are really interesting applications of the fact that language models absorb bias for looking across larger text sets with questions that historians and communication scholars might have about those texts. That’s very different from reinscribing phenomena because it’s used as a lens on the past and asking questions about the past. The other thing that I want to point to is work by Timnit Gebru, et al, looking at how the practices of archiving and data curation that come from these fields can be informative sources for people who are interested in building datasets for machine learning with a better culture of care.
Q: If you take any language model and you divide the number of words that you are using per parameter of the model, this number is going down. At some point you will get to overfitting. What do you think about that?
EB: My specialty is not in the design of machine learning models, so maybe I’m not the right person to ask. But something about the architectures of these models allow them to use their “over-parameterizedness” in ways that doesn’t overfit. (I don’t know if it’s architecture or
training regime.) It seems to be that they don’t run into the overfitting problems that, say, an N-gram model was doing. At the same time they are very finely fit to things in the training data and oftentimes to things that, from a human perspective, are not the important things about the training data. That’s where you get all of these shortcuts and cheats and these problems with our evaluation regime.
Q: Have you seen any papers or any research that you think is heading in the right direction?
EB: Yes, a lot. I think that, in general, what I look for in research is something that is situated and contextualized and has a defined research question, where that research question is appropriately grounded in the world. “Appropriately grounded in the world” could be someone doing pure math and the research question is grounded in other math, and that’s fine, but when the research question is motivated by something along the lines of helping people in a certain situation, well then I really want to see the context: Did you talk to those people? What’s the understanding of the situation? How does this research fit into it?
So I guess the metric that I’m coming around to proposing here isn’t so much framed as a loss metric but as a meta evaluation of research. To what extent is the question asked and the solution proposed? Are they both grounded in the particular motivating case versus abstracted away? And that tends to push towards specificity rather than generality, which is really swimming upstream. You see this in design, linguistics, computer science, where the highest prestige work is high prestige because it can claim generality. It can claim to be more foundational than everything else, and I think that the more you try to be foundational, the more you’re accountable in terms of figuring out how it’s going to behave in the world. I think really solid work tends to be specific enough that it can get its arms around those questions.
Q: I think I read your paper as a plea to get back to actually trying to understand language rather than pretending to understand language with large language models. Since your paper was published, have you seen any move in research groups or student interest towards this?
EB: I get to talk to a lot of people who are interested in other aspects of the problem, other than just trying to get the highest score on the leaderboard. Nothing yet has struck me as a big change in funding priorities. I would love to see that but I haven’t seen it yet. Certainly, since we published this, there have been more and bigger language model things coming out from big tech. Not that we would have hoped to change the course of things instantly upon publishing, even with the weird platform that Google gave us. The best I can hope for is that this conversation has made it normal to talk about. Instead of everyone just accepting ever-larger language models as an inevitable path we’re going down, maybe there’s room to talk about other things.
Q: Considering that these platforms are basically always, to some extent, distributed by a small group of individuals out to many users, how do you know when you have enough of that local input before it’s ethical to scale the system?
EB: And also whose responsibility is it to be testing that? I think there’s a lot more work that we could be doing around licensing. If somebody wants to build something that’s meant to be foundational and scaled across many different contexts, what guidance needs to be given to the person who’s doing something in their own particular context about how to do that testing? If you compare what we do in natural language processing to most other kinds of engineering, we are weirdly unregulated. If we are providing things that become components of other models, how do we provide enough information to the user that they can decide whether or not to deploy it, or what modifications or what tests do they need before they can make that decision?
Q: Like faces that are used on the web for training — for example, facial recognition — do you think the text needs consent? Let’s say I write something on a blog about computer science. Should I give consent to someone to use my text to train these models?
EB: What is the issue for consent for scraped language models? My feeling on this is, to the extent that we can use text with consent, that is better. I think that should be the default. There are cases where you might want to collect and study text without user consent; here I’m talking about work looking at what happens in extremist recruiting discourse online. You’re probably not going to get the consent of the people who created the text, but it seems to me that, at that point, if you’re collecting text without consent for a specific reason, then maybe you don’t just distribute it broadly. Maybe it only gets redistributed to somebody who’s working on a similar topic. Otherwise, just scraping large quantities of text and using them willy-nilly does not seem to meet with best practices from many different research fields that work with text.
Q: Do you think text can be used in a way for some professional barometrics?
EB: I think we need to be equally careful here, and I think it might be a bit harder to remember to be careful. When we’re doing sentiment analysis, we don’t have direct access to what the person writing the text was feeling. The best we can say is we can train a system that agrees with how humans interpreted this text with respect to feelings. Certainly around the question of, “Is this person xyz personality trait?” I think we need to move very carefully.
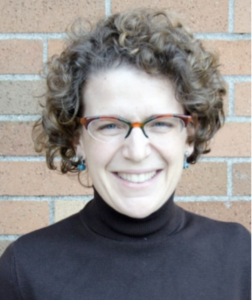
About Emily M. Bender
Emily M. Bender is an American linguist who works on multilingual grammar engineering, technology for endangered language documentation, computational semantics, and methodologies for supporting consideration of impacts language technology in NLP research, development, and education. She is the Howard and Frances Nostrand Endowed Professor of Linguistics at the University of Washington. Her work includes the LinGO Grammar Matrix, an open-source starter kit for the development of broad-coverage precision HPSG grammars; data statements for natural language processing, a set of practices for documenting essential information about the characteristics of datasets; and two books which make key linguistic principles accessible to NLP practitioners: Linguistic Fundamentals for Natural Language Processing: 100 Essentials from Morphology and Syntax (2013) and Linguistic Fundamentals for Natural Language Processing II: 100 Essentials from Semantics and Pragmatics (2019, with Alex Lascarides).