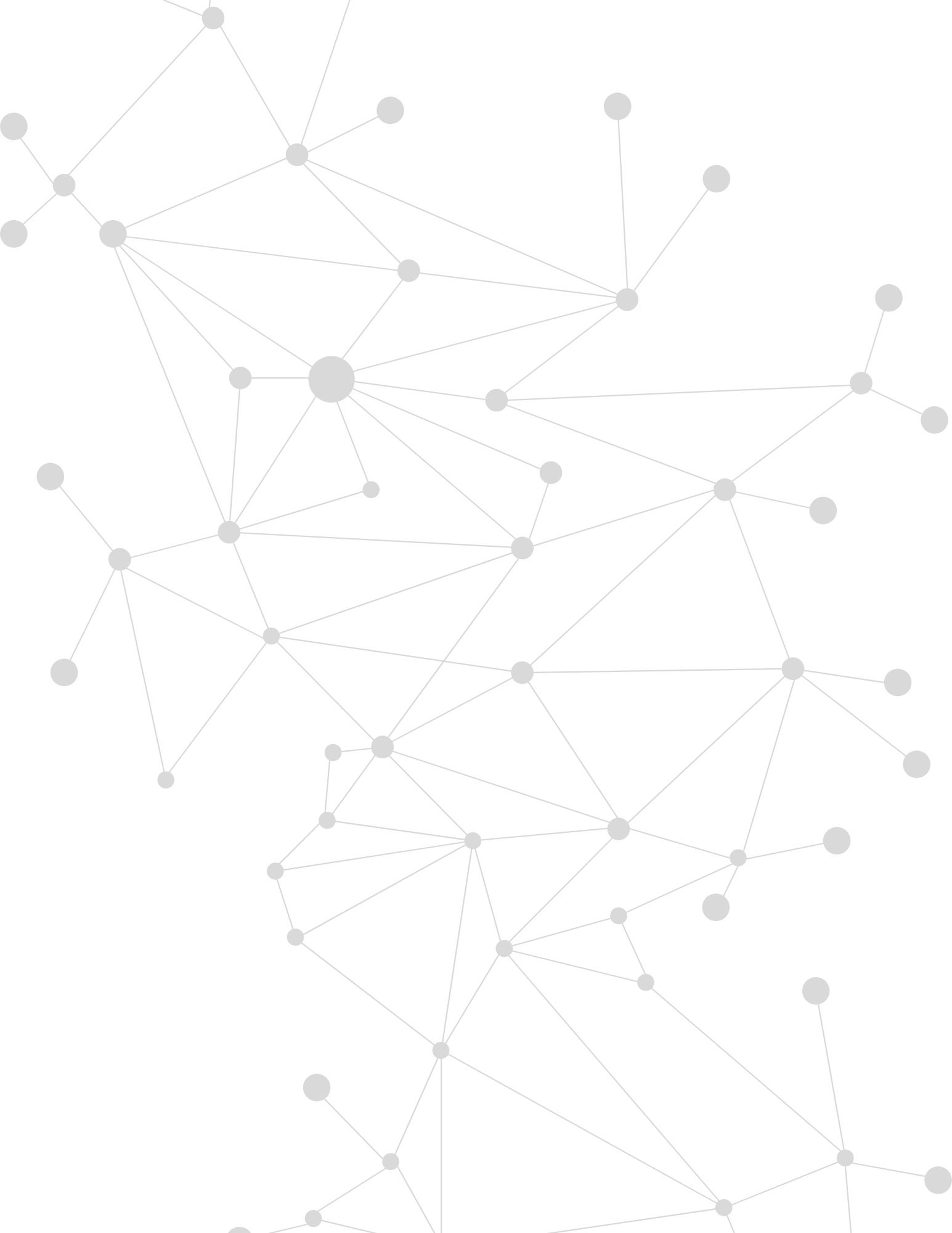
An Automatic System to Detect Equivalence Between Iterative Algorithms
Large-scale optimization problems in machine learning, signal processing, multi-agent systems, and imaging have fueled ongoing interest in iterative optimization algorithms. New optimization algorithms are regularly proposed in order to capture more complicated models, reduce computational burdens, or obtain stronger performance and convergence guarantees.
But how can we be sure a recently proposed algorithm is novel?
Algorithms can be written in different equivalent ways that are not always obvious, and with optimization being increasingly prevalent across different applications, popular algorithms are routinely "re-discovered". In this talk, we present a framework for reasoning about equivalence of iterative algorithms.
Our framework is based on concepts from control theory and linear systems theory and can identify equivalence for a variety of algorithm classes:
(a) single-oracle algorithms such as gradient-based methods
(b) multi-oracle algorithms such as distributed optimization algorithms, primal-dual methods, and operator-splitting methods, and
(c) algorithms that use different but related oracles, such as subdifferentials, proximal operators, and Fenchel conjugates.
Our work is a promising step towards an integrated and principled methodology for analyzing and designing control systems that use optimization algorithms "in the loop".
Keynote and Industry Speakers
Northeastern University Speakers
Agenda
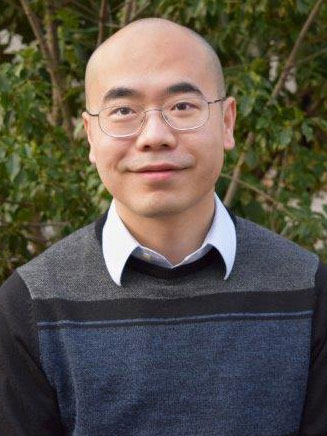


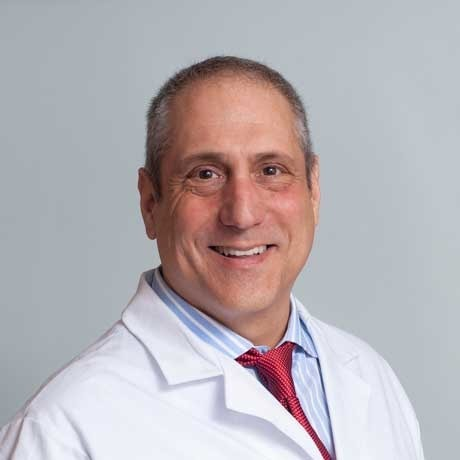
.png)
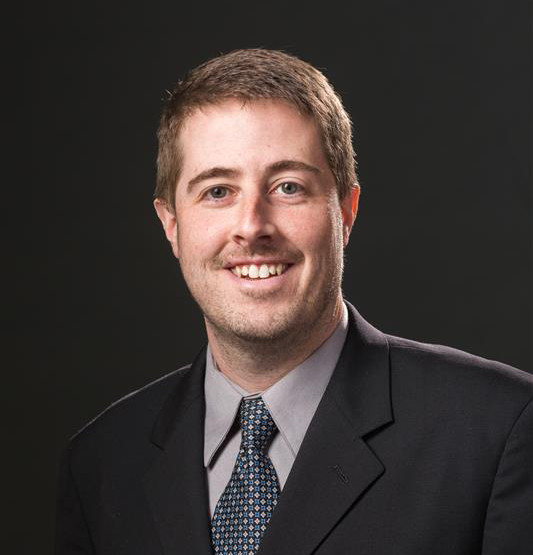
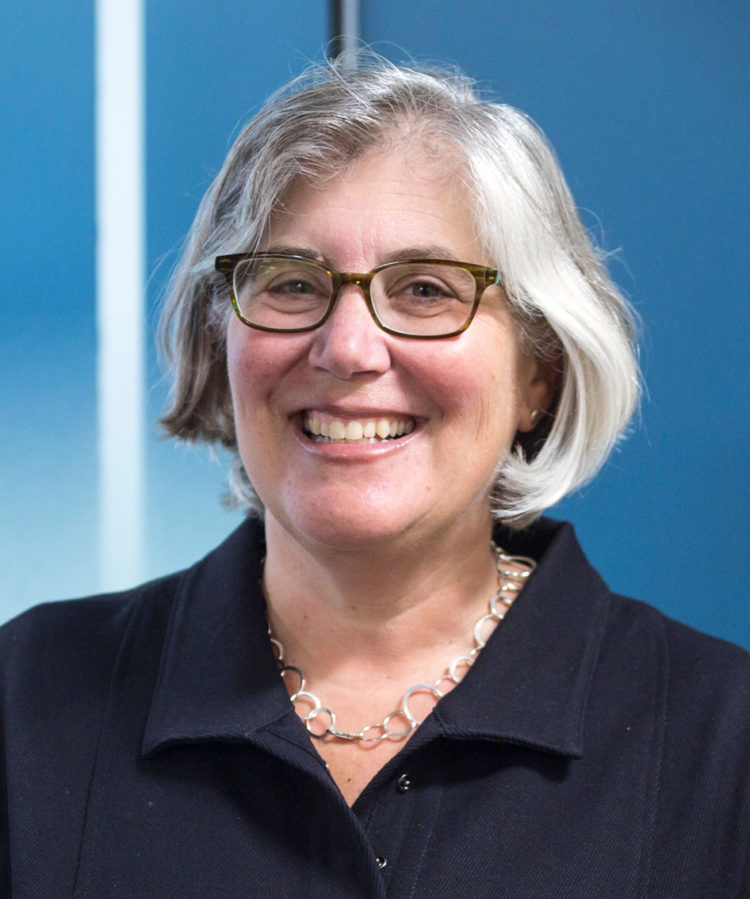
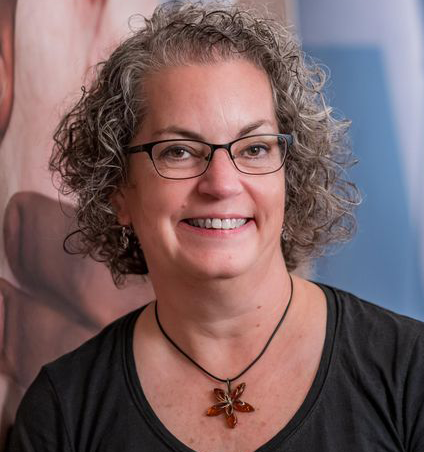
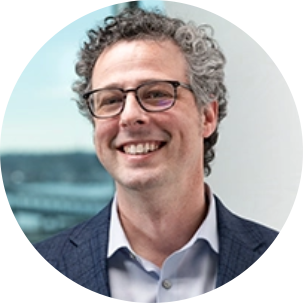

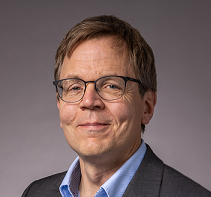
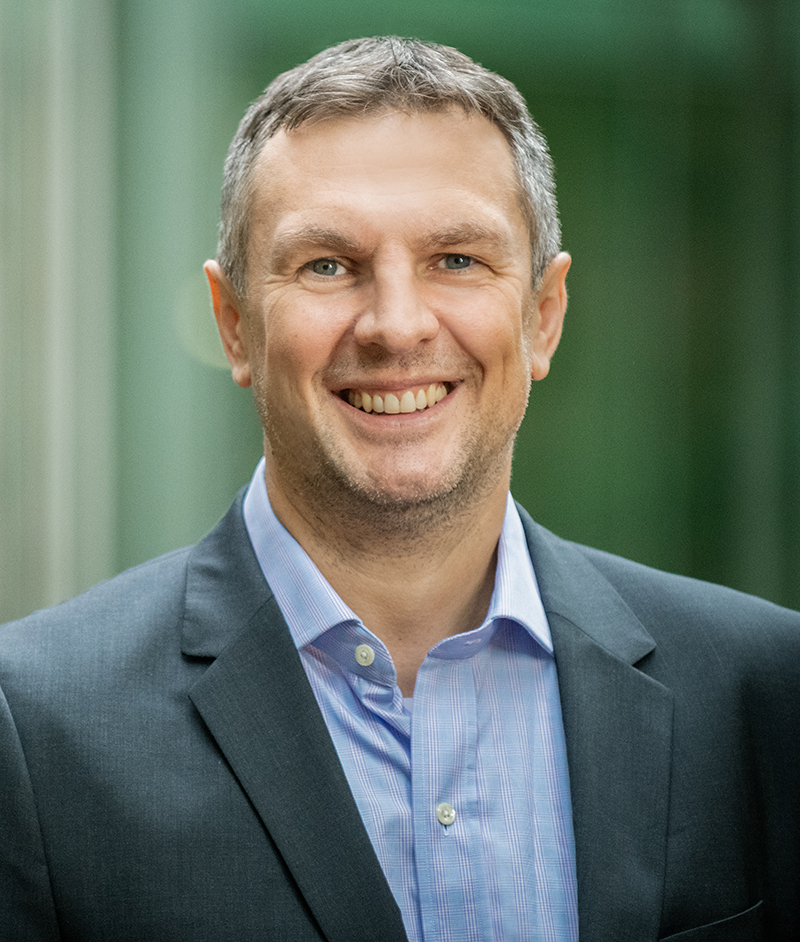
%20circ.png)
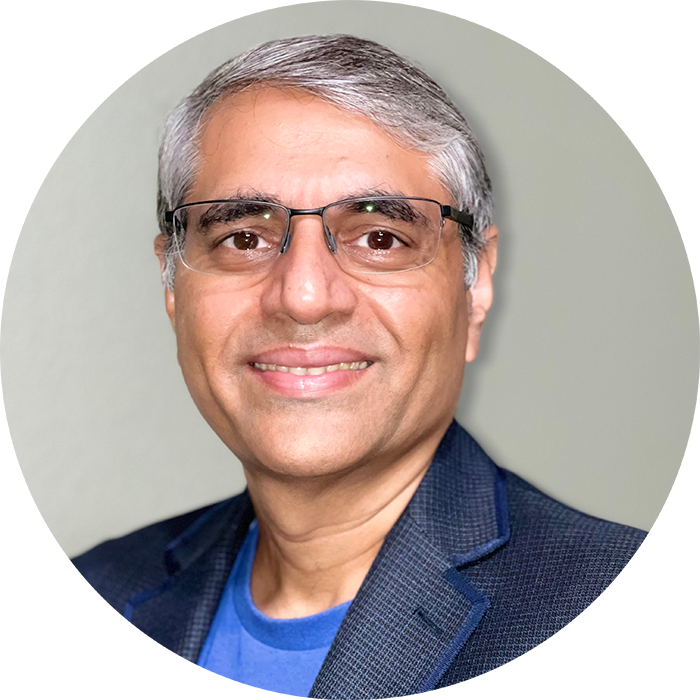