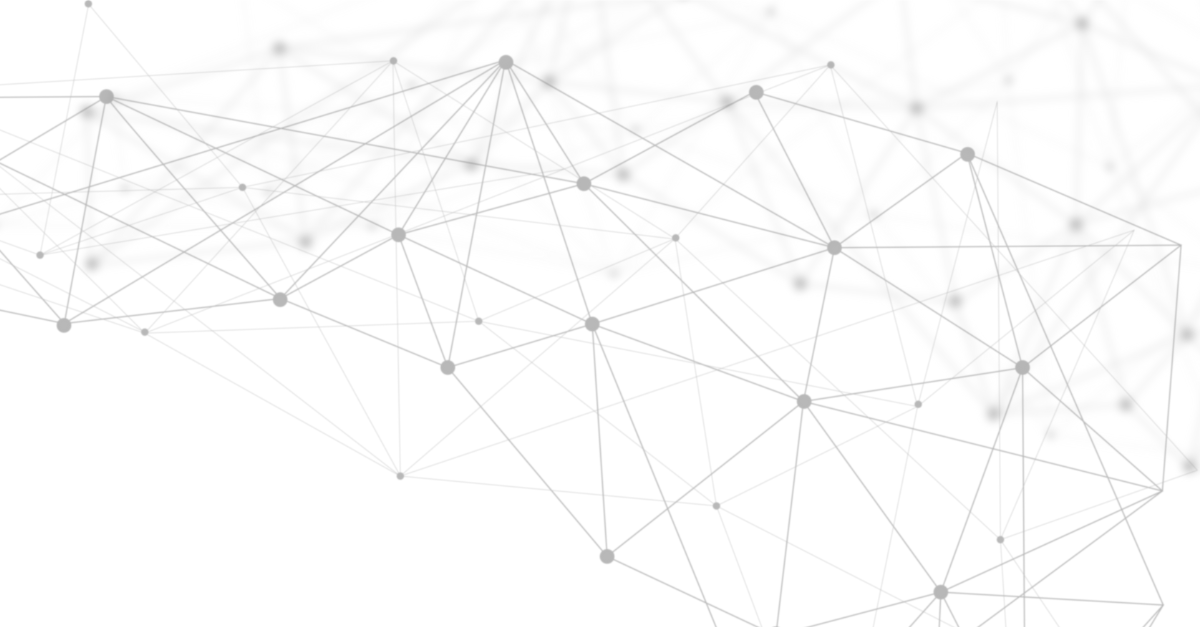
Abstract
Data-driven materials science often titled the “4th paradigm”, has opened an avenue towards material discovery through statistically driven machine learning approaches. Due to the continued increase in computing power and improvements of theoretical methods, the accuracy of predicted material properties has reached reliability comparable to experiments while significantly surpassing them in terms of speed and cost. This gave rise to a rapid increase in available open-source material databases, facilitating material discovery at an unprecedented pace.
In this talk, Peter describes how a data-driven approach can facilitate discovering new materials with tuned properties and how AI can be a powerful tool to augment (however not replace) our physics/chemistry-based intuition. To illustrate these concepts, he describes how his research enables the discovery of new ultra-low work function materials for thermionic energy conversion. He demonstrates that creating data by high-throughput quantum chemistry calculations forms the basis on which a statistically driven machine learning model can be established. This model can then predict the property of interest with an accuracy comparable to the first principles calculations while being significantly less computationally expensive. Further, Peter provides his perspective of where he sees the machine learning paradigm in materials science and sustainable energy in the near future.
Flip through the slides from the presentation here:
Dr. Peter Schindler is an assistant professor at Northeastern University (Department of Mechanical & Industrial Engineering). He’s leading the Data-Driven Renewables Research (D2R2) group that seeks to discover novel materials for renewable energy applications using high-throughput, quantum chemistry calculations, and data-driven materials property predictions. Prior to joining Northeastern he was a senior scientist at Aionics, a startup that has become a recognized leader in the field of battery informatics, by co-innovating with battery manufacturers through AI and high-performance computing.
Read more about Peter here.
Keynote and Industry Speakers
Northeastern University Speakers
Agenda
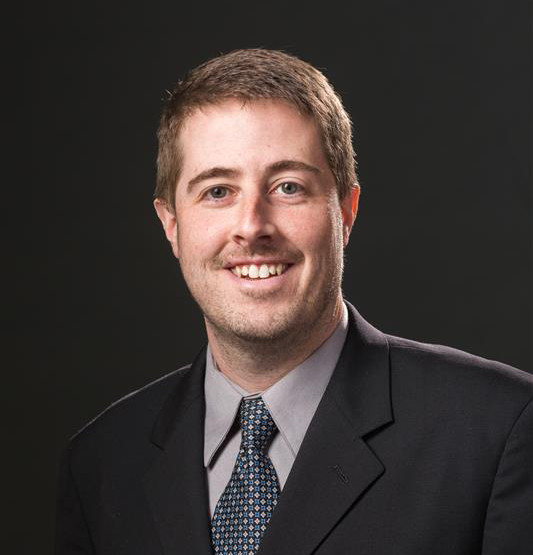
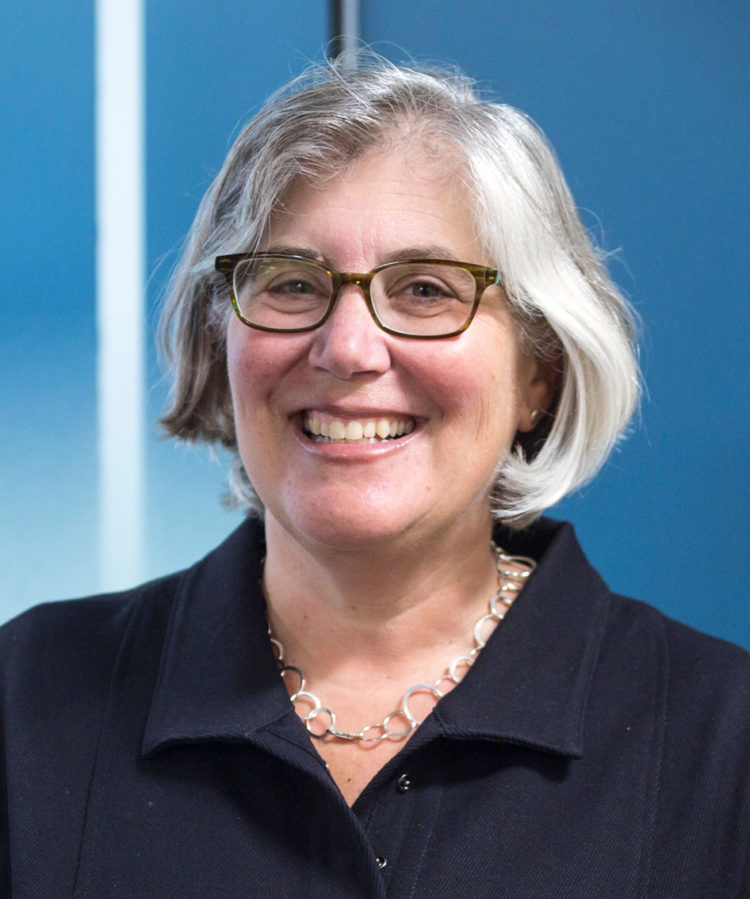
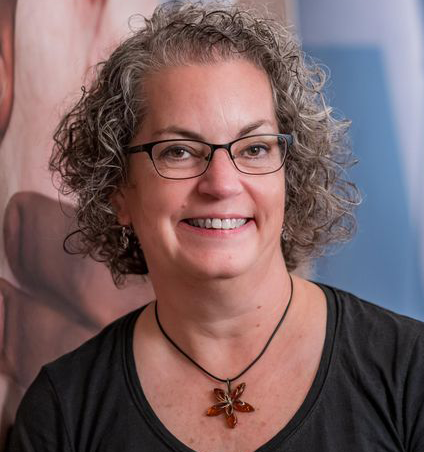
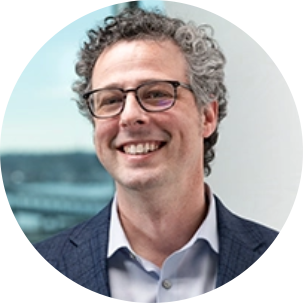

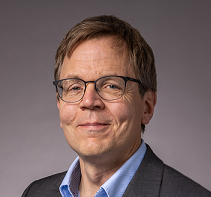
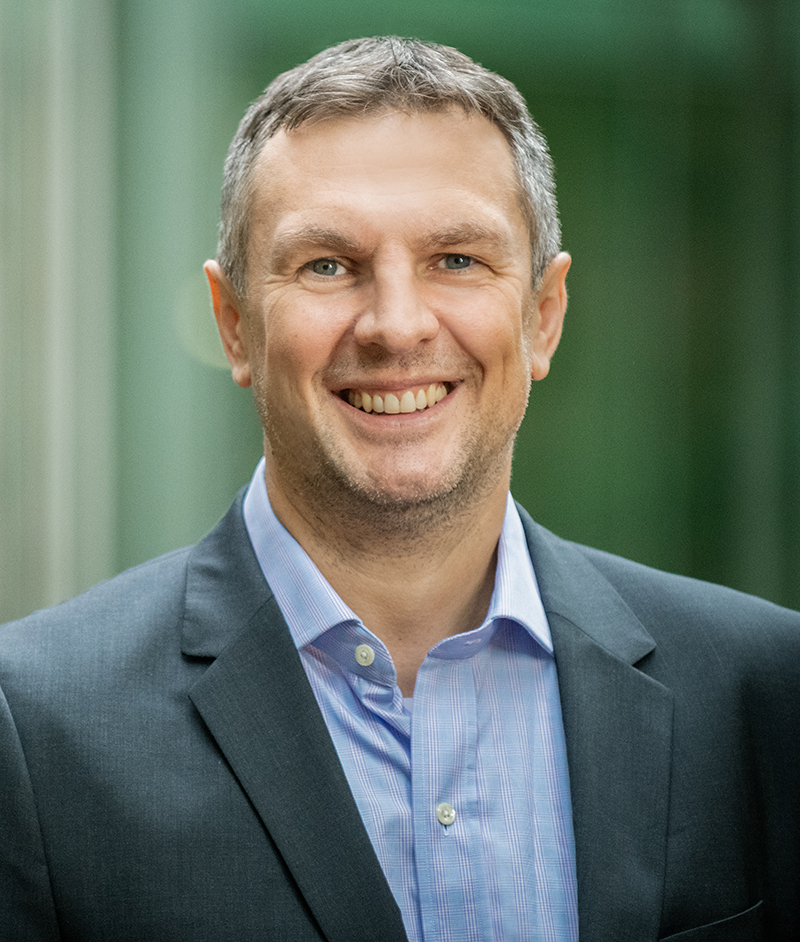
%20circ.png)
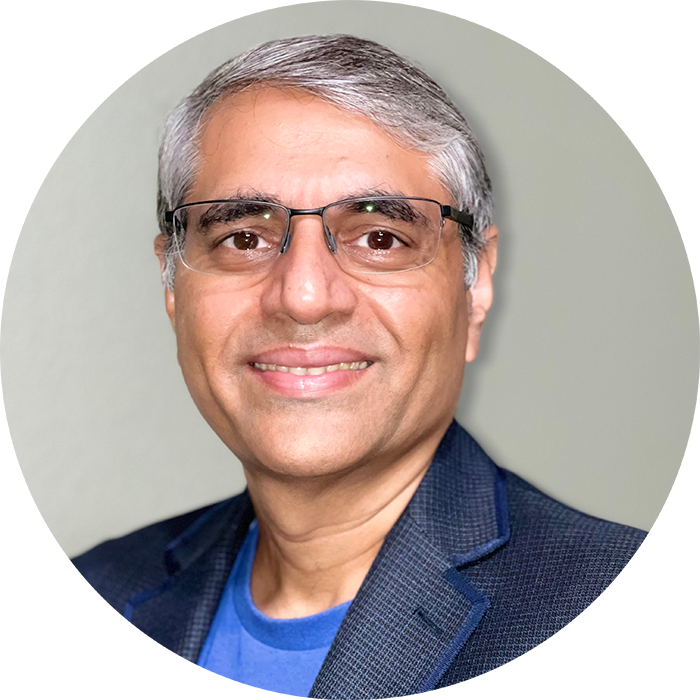
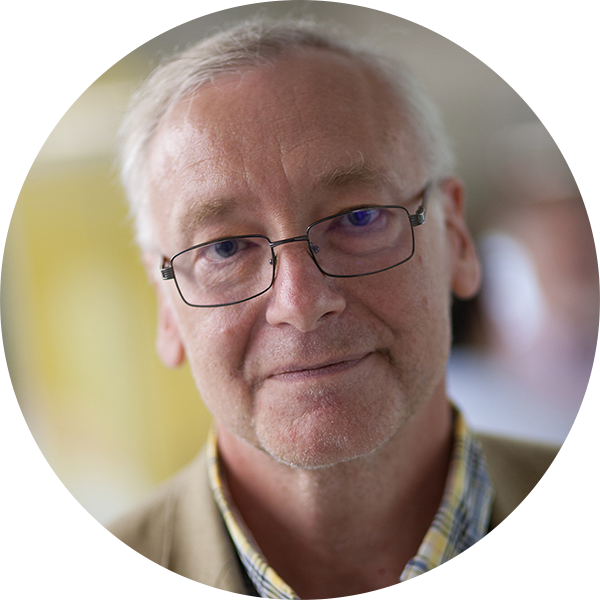
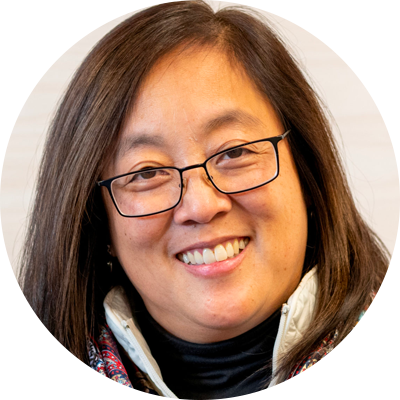