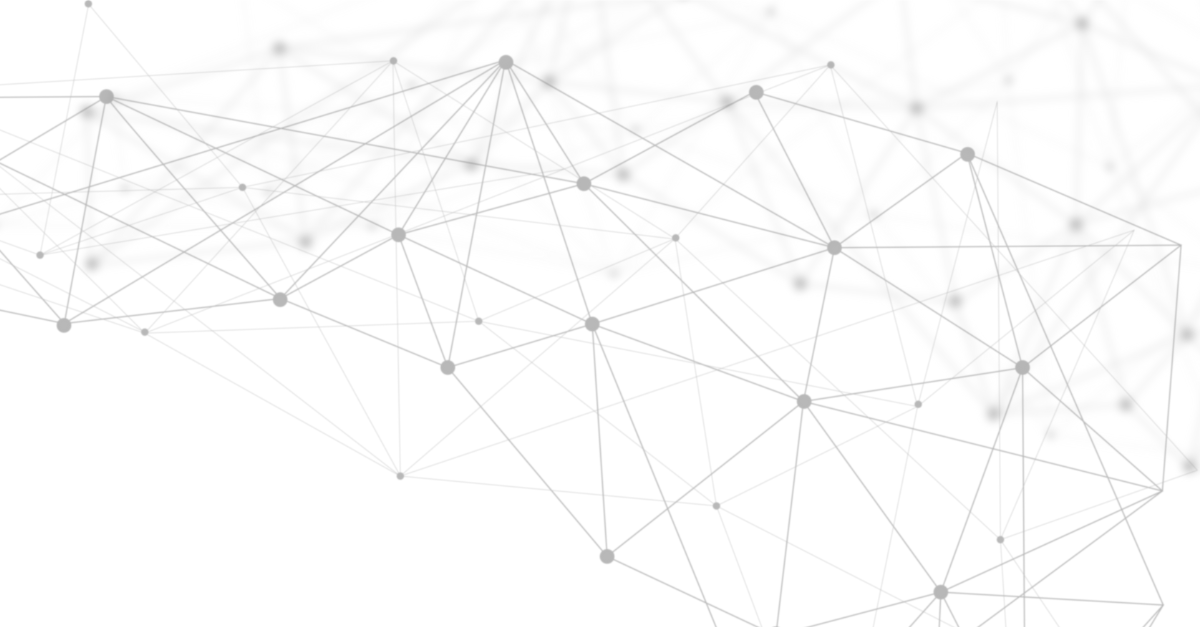
The Three Approaches to Unlearning
Expeditions in Experiential AI seminar with David Bau
Abstract:
What recourse do we have when machine learning models learn something that they should not? In this seminar, we will survey the technical approaches to unlearning through data curation, training, and inference, and we discuss the current state-of-the-research in erasing undesired knowledge from the parameters of large pretrained models. We will focus on the problem of erasing specific knowledge from generative language models and image synthesis diffusion models that have been abused for their ability to create CSAM, copyrighted content, WMD information, and other undesired capabilities. Finally, we touch on some of the ecosystem and policy challenges posed by unlearning.
Bio:

David Bau is an assistant professor at Northeastern University Khoury School of Computer Sciences. He is a pioneer on deep network interpretability and model editing methods for large-scale generative AI such as large language models and image diffusion models, and he is author of a textbook on numerical linear algebra. He has developed software products for Google and Microsoft, and he is currently leading an effort to create a National Deep Inference Fabric to enable scientific research on large AI models. He received his PhD from Massachusetts Institute of Technology, his MS from Cornell University, and his BA from Harvard University.
Keynote and Industry Speakers
Northeastern University Speakers
Agenda
.png)
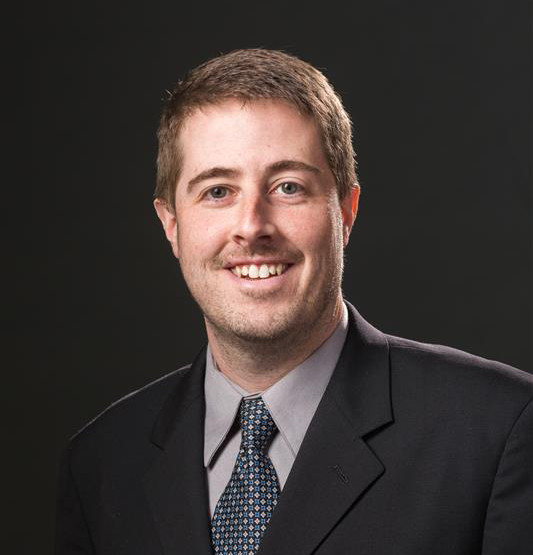
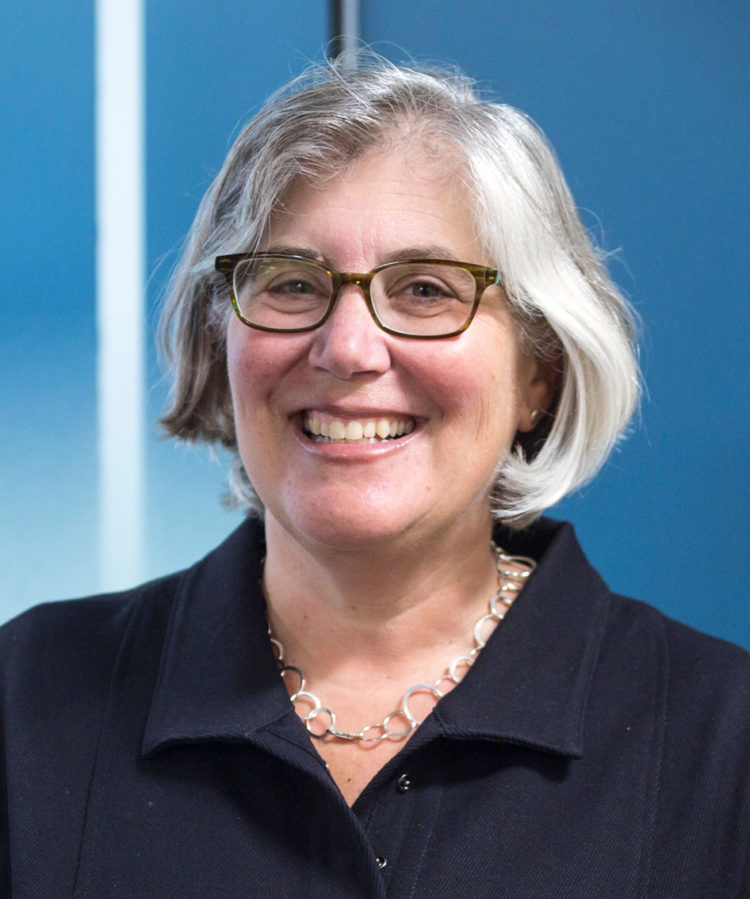
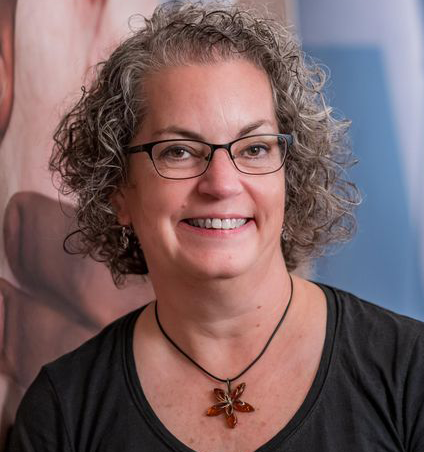
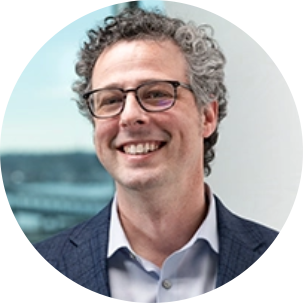

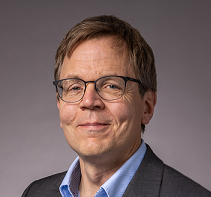
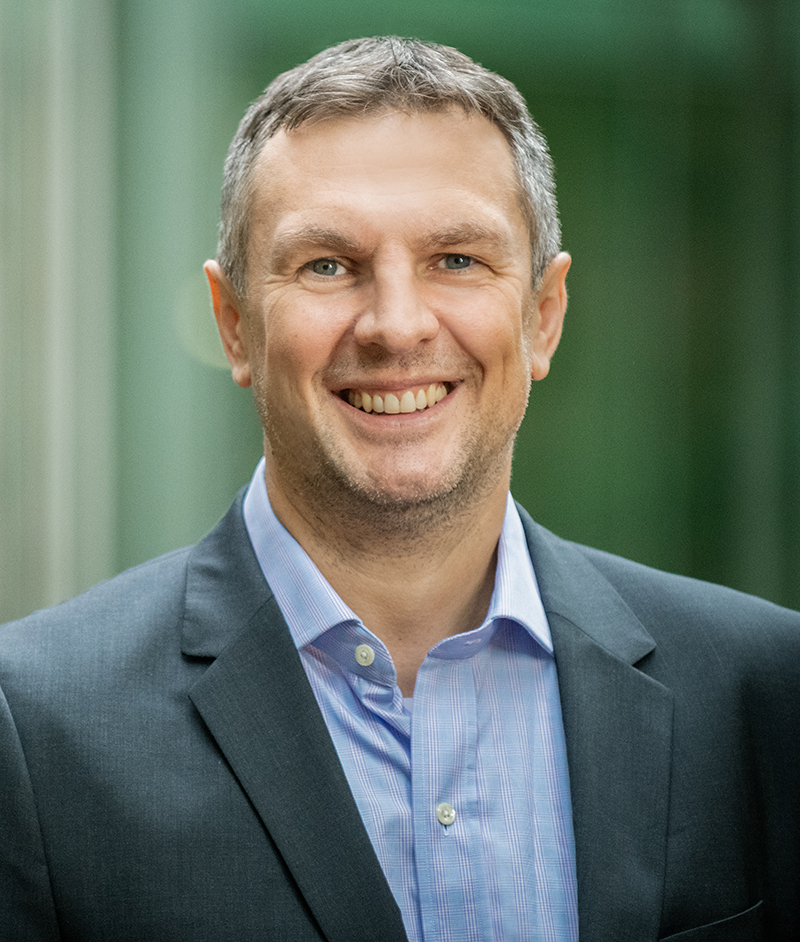
%20circ.png)
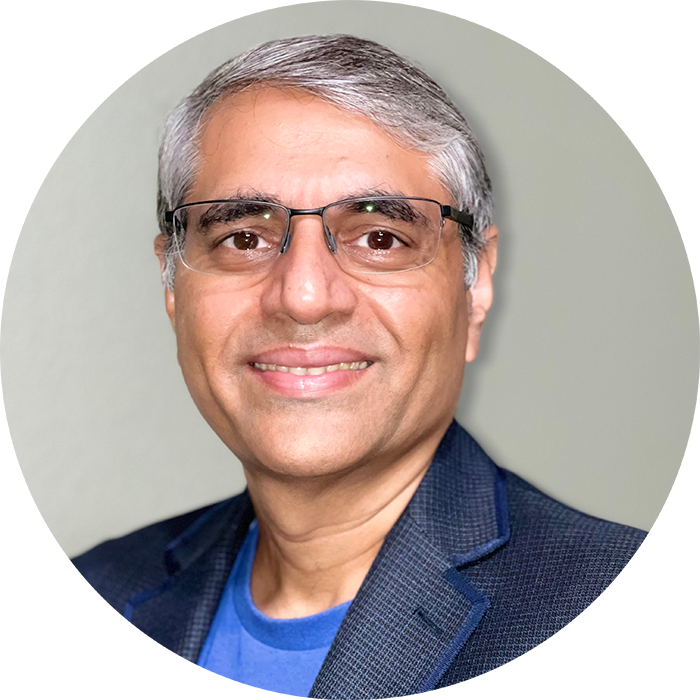
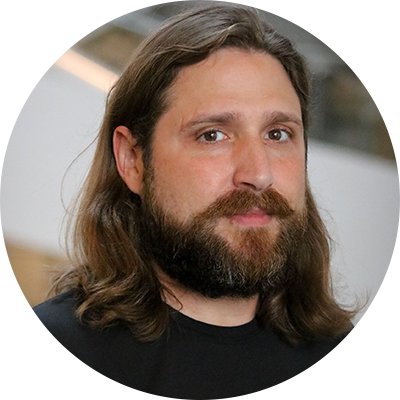
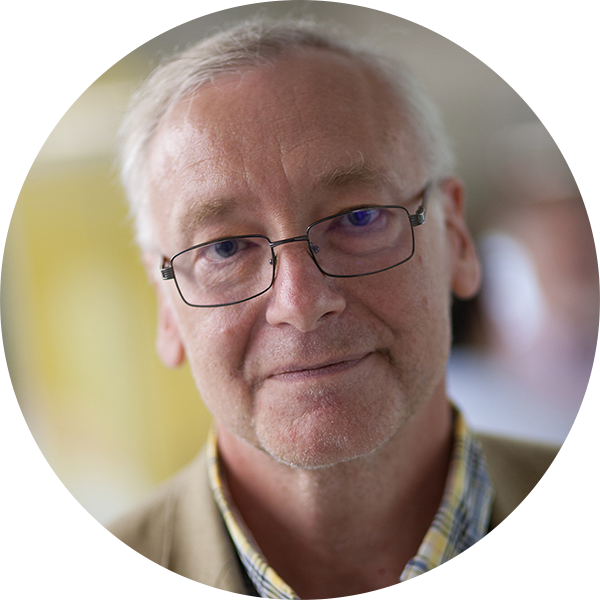
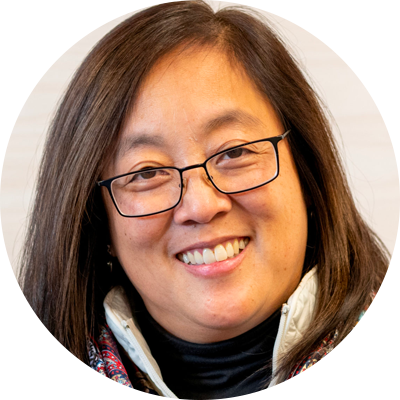