Four Ways to Create an AI-Driven Organization
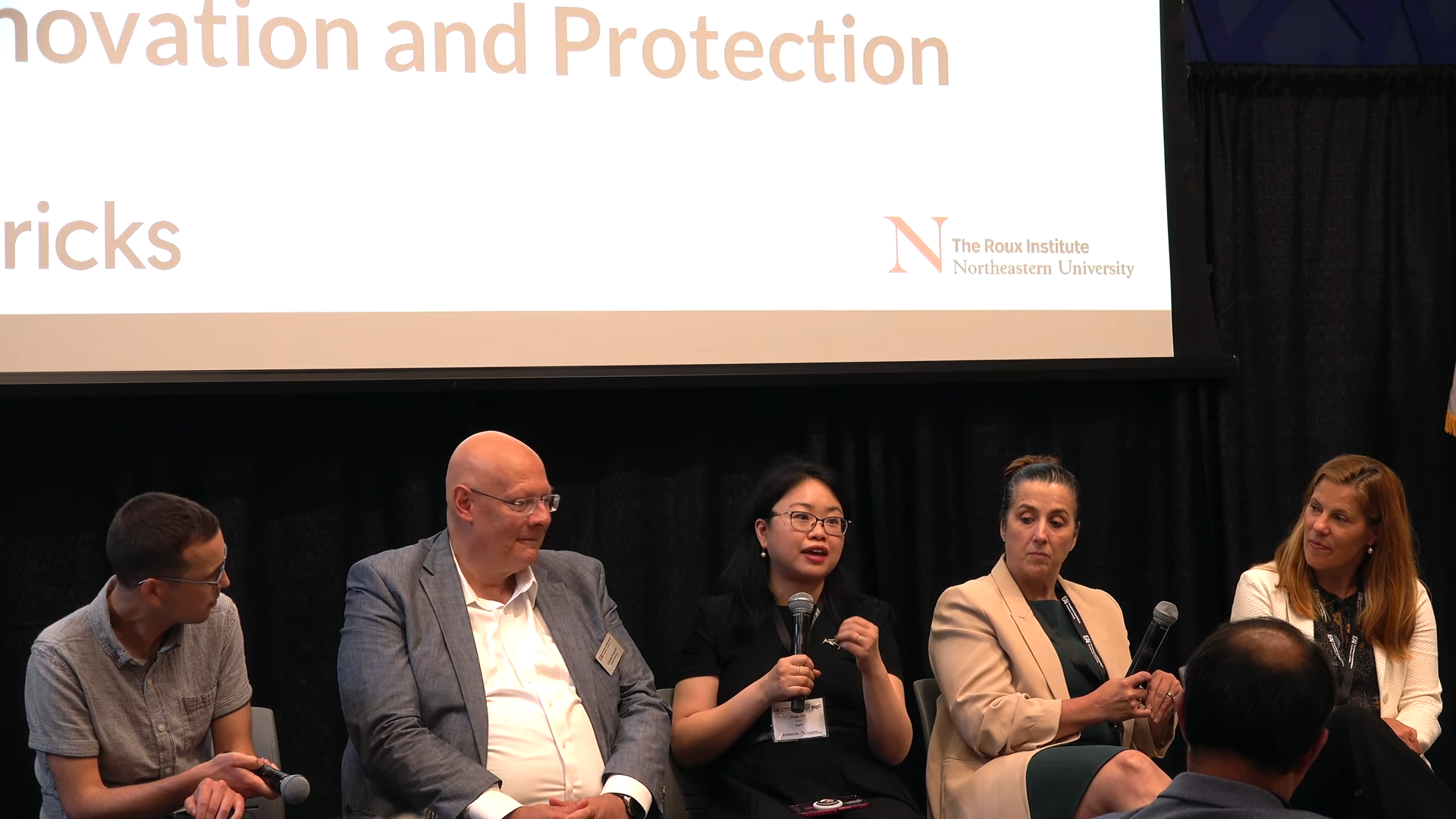
“What’s our AI strategy?”
The question gets passed down from boards to C-Suites to managers in every corner of an organization. But no single person can harness AI to its fullest effect in isolation, and top-down mandates won’t work unless executives spend time thoughtfully investing in the data infrastructure that makes or breaks AI solutions.
Challenges related to adopting AI were among the most discussed topics at a recent gathering of high-level executives at Northeastern University hosted jointly by BigID, the Institute for Experiential AI at Northeastern University, and DataBricks.
One of the organizers of the event was BigID Chief Data Officer Peggy Tsai. Through her role with BigID and nearly 20 years working in data roles on Wall Street, Tsai has collaborated with hundreds of executives to improve their data and AI strategies, and she’s come to recognize patterns that lead to success.
Tsai’s broad expertise aligns with that of the leaders at the Institute for Experiential AI, who work with leaders across various industries, including health care, energy, and finance, to execute AI strategies tailored to their specific needs and goals.

Below we share some broad themes shared by event attendees, Tsai, and members of the Institute for Experiential AI.
1. Building AI Competency from the Ground Up
The success or failure of an AI investment is determined long before a company starts building models. Companies that want to get the most out of AI need to invest in educating and training a broad swath (if not all) of their employees on how AI works and what it can do.
Employees should also be trained on basic data literacy. Tsai suggests fitting data training into standard employee onboarding processes. She also says organizations should invest in tools that allow employees to analyze and manipulate data on their own. None of this is easy.
“It takes years of investment and work to set that foundation,” Tsai says. “But once you have that foundation, any employee can easily look at a dashboard and apply basic filters to analyze the sales or engineering or marketing information they need to understand to do their jobs more effectively.”
2. Name an AI Champion
Every employee has a role to play in helping their company leverage data and AI, but decisive action — not to mention accountability — also requires clearly defined ownership and responsibilities for AI projects.
If CEOs are hesitant to make a single person responsible for their company’s AI strategy, they should at least name an “AI champion” with the authority to cut across traditional divisions to align their company’s data infrastructure and processes with AI’s strengths. Tsai says this person should report directly to the CEO and have as much respect in an organization as traditional C-Suite positions like chief technology officers and chief finance officers.
One increasingly common role being created is chief data officer or chief AI officer. Those are among the newest roles in the C-Suite. In fact, Institute for Experiential AI Executive Director Usama Fayyad became one of the first CDOs when he assumed the title at Yahoo in 2003. Fayyad recently spoke on a panel at the annual CDOIQ event about what makes a CDO successful, and many of his idea were echoed by Tsai.
The ideal CDO, according to Fayyad and Tsai, is a team player able to collaborate across an organization. They must work well with others in the C-Suite as well as coworkers on the technical side, compliance officers, and sales, marketing, and business development.
“Good chief data officers need to be influencers,” Tsai says. “CDOs may need to convince people across the organization to do things they don’t want to do. CDOs also don’t always have the IT resources or staff to do things like clean data, so they need to enlist other teams to help and align other groups in the organization.”
3. Treat Data As a Primary Business Asset
In the age of AI, data is king. As Fayyad often says, “No data, no AI.” That means data governance and data quality need to become a first-order priority for companies interested in tapping into the power of AI. This is especially true with the emergence of generative AI and large language models (LLMs).
“The ROI of these generative AI projects is not going to be realized without good data,” Tsai says. “Thus far, many companies applying standard LLMs on top of their company have only seen mediocre results in terms of accuracy. The success of generative AI hinges on data governance, data quality, and data management.”
That might require a shift in mindset as people learn to tie high-level business goals and outcomes with data.
“Your CDO [or other AI champion] should take the CEO’s vision and identify down to the table or column-level the datasets that can be used to support that vision,” Tsai says. “Data storytelling is so important. One of the reasons CDOs are ignored or not given a seat at the table is because they haven’t been able to articulate the value of data to their peers or the CEO.”
4. Know When to Build and When to Outsource
Anyone who’s been paying attention to recent earnings reports from the likes of Microsoft, Facebook, and Google understands that building generative AI models can be expensive. Big tech companies are investing billions of dollars into generative AI — expenditures that would put most organizations out of business.
Organizations thus need to do think carefully about how they invest in building and implementing AI in-house versus using external tools. There is no one-size-fits-all solution. The right approach depends on company-specific factors such as data and organizational structures, the number of employees with AI expertise, capital constraints, and more.
One way to make these decisions is to partner with outside experts. The Institute for Experiential AI’s AI Solutions Hub (AISH) works with businesses to help them evaluate the highest-ROI opportunities through our Data Opportunities Roadmap. The AISH also offers an AI Ethics Advisory Board to help executives navigate the AI landscape and has extensive experience building tailored AI solutions for organizations. The services are designed to help businesses stay lean while reaping the benefits of a cutting-edge technology.
AI is one of the fastest-evolving fields of our time. The changing technological landscape requires organizations to be strategic: An in-house AI project that takes two years to build may be embarrassingly dated by the time it gets to market.
From Planning to Executing
Business leaders are right to be skeptical of the wild hype surrounding AI today. But underneath that hype are powerful capabilities that, if harness correctly, can drive real business value. Fayyad estimates AI will accelerate knowledge worker tasks by 15 to 80 percent. Tsai also sees AI’s role in businesses growing over time.
“This isn’t something that will go away,” Tsai says. “AI has permeated not only the technology space but every industry and sector. Everyone is trying to use it right now, even consumers. Companies can’t afford to walk away from AI solutions.”
And as AI technology matures, its impact on the business world will only grow. The companies that ride the advancement of AI will build a moat around their businesses and replace the companies that don’t.
Businesses can become paralyzed trying to answer the question “What’s our AI strategy?” Tsai believes another question may be more apt:
“The next wave of AI use cases will be even more seamless and intelligent in its ability to help employees and customers find value,” Tsai says. “The biggest question for every organization is really, ‘How can we keep up?’”
Want help solving your organization’s data problems? We partner with organizations to solve pressing data problems and harness AI to deliver business value. Our team of leading data scientists, AI researchers, practitioners, and Northeastern University faculty members apply the latest methods and techniques to tackle challenges at the pace of innovation.