AI and the Environment: Is It Enough to Lead by Example?
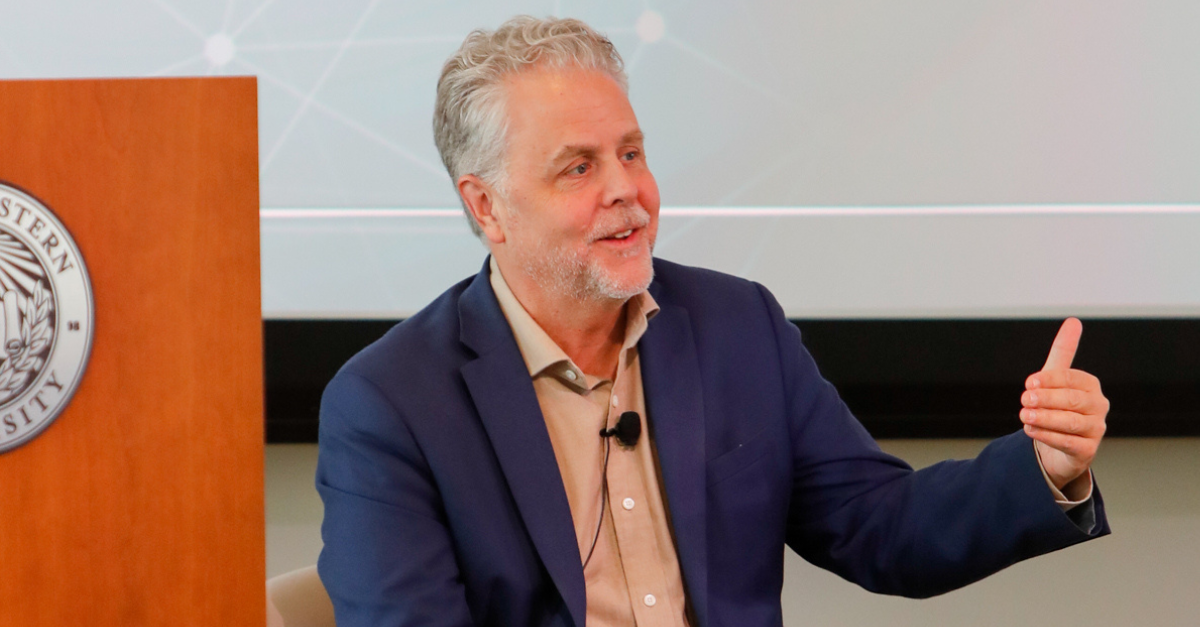
AI systems are nothing if not power hungry. Researchers from the University of Massachusetts found training just a single AI model can emit the same amount of carbon dioxide as about 63 gasoline-powered cars driven for a year. Another study estimated that emissions from the Information and Communications Technology (ICT) industry as a whole will reach 14% of global greenhouse emissions by 2040.
Delineating carbon emissions back to the design stage of individual products for the purpose of minimizing climatological impact is more than just a technical challenge—it’s an ethical quandary. Incentive structures, specifically when it comes to legal responsibility, are rarely aligned in favor of the environment. Even the way we talk about AI’s impact rarely accounts for the wellbeing of the planet. But maybe that should change.
At Leading With AI, Responsibly, Peter Norvig, Google researcher and fellow at Stanford HAI, defined human-centered AI as AI that engages diverse, multidisciplinary teams early in the development cycle with the explicit goal of examining and anticipating the broader impact of a system. There’s no reason why that “impact” shouldn’t include the planet.
Ignorance Is No Excuse
The problem is that AI’s environmental footprint tends to scatter and cloak itself in the murky business of complex networks and systems, making it harder to identify where and how AI is causing harm. John Havens, director of emerging technologies and strategic development at the IEEE Standards Association, made a passionate plea illustrating just how messy these questions can get:
“Ignorance is not an excuse for irresponsibility,” he said in a sideline interview. “When you build something like a car in North America, you may think, ‘I’ve done my job with ESG transmissions and know my emissions are not going to harm this nearby area,’ but you don’t necessarily know that the car parts you’re making, if they go into the ground, are going to cause a form of pollution that may affect a global supply and value chain.”
When it comes to AI, the “value chain” hardly accounts for climate impact as is. Companies don’t need to disclose their energy usage for AI systems (and rarely do), and the resources consumed are not only the electricity used to power computers. One recent study estimated that training GPT-3, the large language model behind ChatGPT, might have consumed as much as 700,000 liters of fresh water just to cool its data centers.
Leading by Example
It’s worth asking: How can a Responsible AI framework begin to account for such a frenzy of consumption? And how, given the regulatory desert that is the AI industry in the U.S., can anyone expect developers to lead with global human and planetary considerations?
If you were to ask some of the guests at Leading With AI, Responsibly, they would probably tell you to lead by example. Philip Brey, a professor of philosophy at the University of Twente, stressed in a panel on the practice of Responsible AI, that, “If from the outset you prioritize the ethics that people embrace, then products and services will meet with less resistance.”
Xuning Tang, associate director of Responsible AI at Verizon, agreed, but also stressed the need for quantifiable metrics: “What’s lacking is how to measure or assess yourself in terms of the maturity of your AI governance program. Only if you can measure yourself can you know if you're moving in the right direction.”
John Havens, for his part, took a more radical approach, arguing that the myopic focus on growth and productivity comes at a cost to the ideals that make life worth living for all of Earth’s inhabitants, questioning the assumption that “slowness” is inherently bad.
“The question is, what do we want with our humanity?” he asked. “Is it to make sure our kids grow and flourish whoever they are around the world in a planet that can sustain us for generations? Then let’s remove the constant barrage of GDP-centric pressure that has led us to the anthropocentric place where we are. Bringing nature into these conversations I sometimes feel embarrassed, but we need nature to live.”
AI + Resilience
For Ardeshir Contractor, director of research for the AI for Climate and Sustainability (AI4CaS) focus area at the Institute for Experiential AI, the environmental role of AI is a matter of perspective. In a lightning talk covering the research priorities of the institute, he explored ways AI can and is being used not only to help scientists understand climate change but also to make communities more resilient to its effects.
His team’s research involves using AI to combine disciplines, such as advanced computational science, engineering, physics, and bio-geoscience. One of the primary goals here is to create new, more robust datasets that reflect the varied and diverse conditions of planetary systems.
“I think the most important thing,” Ardeshir said, “is that we want to create a focus on resilience — the ability for communities, cities, transportation networks, energy outlays to actually be able to weather the extremes that we are going to see because of climate change.”
Every natural system is always in a state of changing—a basic truth that’s not strictly reflected in the historical datasets many AI systems rely on. That’s a problem. And breaking problems down into smaller and smaller components doesn’t always yield the kinds of understandings that scientists or policymakers are looking for. Dynamic systems that convey change are part of the package needed to accurately model environmental conditions as they relate to cities, communities, and transportation and energy networks.
So it would seem the environmental impact of AI cuts both ways: On the one hand, its status as a “resource hog” leaves something to be desired, and on the other, its capacity to help us better understand what is happening to the planet reflects an indispensable tool. Can we have one without the other? Or is it on us to develop a more nuanced appreciation of technology—warts and all?
Learn more about the AI for Climate + Sustainability (AI4CaS) research focus at the Institute for Experiential AI.