Don’t Just Rent AI Capabilities — Build Them.
.png)
A Thoughtful Add-On to Paul Baier’s Case for ChatGPT Teams
Paul Baier, CEO, GAI Insights, in his recent article makes a strong case: leadership teams must get hands-on with GenAI tools like ChatGPT Teams to understand their potential. He’s right — GenAI is a contact sport.
We agree that executive teams can’t afford to sit on the sidelines.
But here’s a complementary perspective: what if that $30K investment was used to build internal GenAI capacity — not just access a platform?
Beyond Tools: Why Investing in Internal Capacity Might Offer Greater ROI
1. Learn the why and how, not just the what.
Training on ChatGPT teaches leaders to use a tool. But what builds long-term strategic advantage is helping them understand when, why, and how to apply GenAI — and when not to.
Take the JFK declassified files as an example — when run through GenAI, even advanced models struggled to make sense of the unstructured content. What it revealed was clear: structured data and internal tooling aren't optional — they're essential.
2. Center the learning on your actual data, workflows, and goals.
Prebuilt prompts are great for inspiration, but organizations see the most value when AI is trained, tested, and deployed on their own processes and pain points.
Your data isn’t clean, neat, or simple and neither are your business challenges. Learning to shape GenAI around your reality is where the payoff is.
3. Build internal muscle, not external dependency.
Tools will evolve. Interfaces will change. What doesn’t change is the need to build a core competency in evaluating, governing, and deploying AI in ways that reflect your values and business model.
As Stephen Klein recently pointed out, relying too heavily on closed-source tools limits your ability to audit, adapt, and innovate — creating long-term risk even as short-term adoption surges.
4. Responsible AI isn’t a feature — it’s a framework.
Even the most secure platforms won’t guide your leaders through bias detection, fairness reviews, or compliance guardrails. That work starts inside.
Reframing the Opportunity
Paul’s plan rightly puts senior leaders in the driver’s seat of AI exploration. We’d just offer this companion view:
Use that same $30K as a launchpad for tailored, organization-specific AI work: strategy sprints, department-based use case development, responsible AI planning, and ongoing capacity building. And importantly: invest in structuring your data and building internal tools that actually work with it.
It’s not either/or — many organizations may start with ChatGPT Teams. But as AI maturity grows, shifting the focus inward will position you for deeper, differentiated impact.
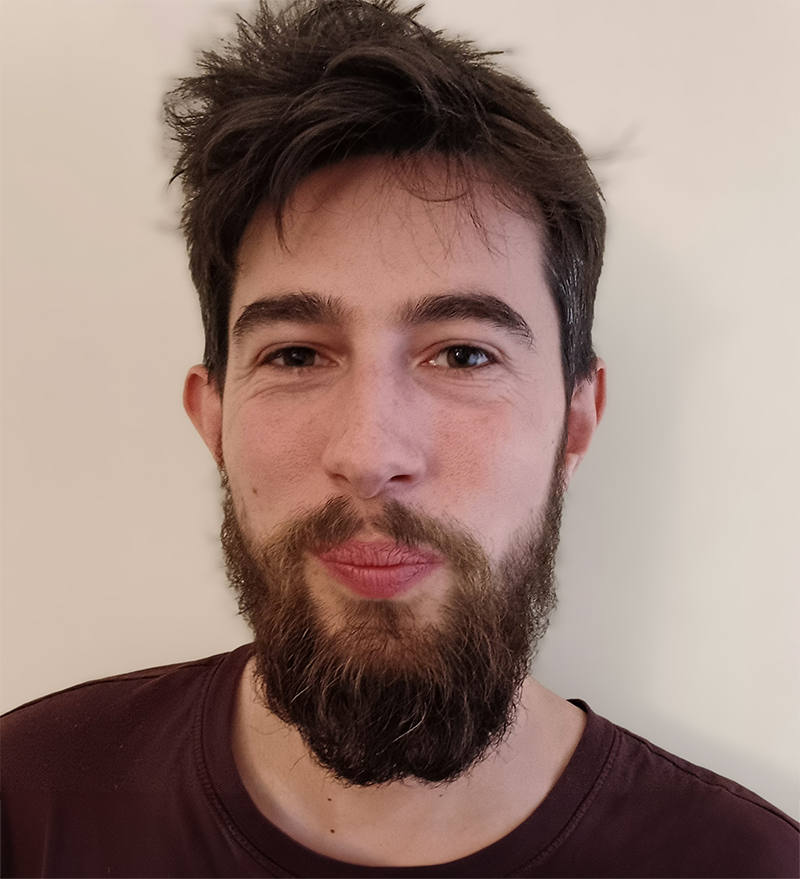
Data Scientist
AI Solutions Hub
LLMs do not solve business problems. Processes solve business problems. While we've seen impressive results with Generative AI across many applications, there is a significant business value amplification when strategy, processes, staff, and technology operate in deliberate alignment. By building AI capabilities in-house, organizations develop the institutional muscle to align technology with their unique processes and challenges in ways vendors simply cannot match.
The Real ROI?
✅ Shared literacy across leadership and your org
✅ Custom use cases with your data
✅ Strategic alignment on AI priorities
✅ Embedded governance and oversight
✅ Momentum for long-term innovation
✅ Tools that reflect your data, context, and evolving needs not just what’s available in the marketplace today
Let’s keep the conversation going. The more we collectively learn, the better we can guide AI’s role in our organizations with confidence, clarity, and care.
.png)