Gary Marcus on Finding a Better Model for Artificial General Intelligence
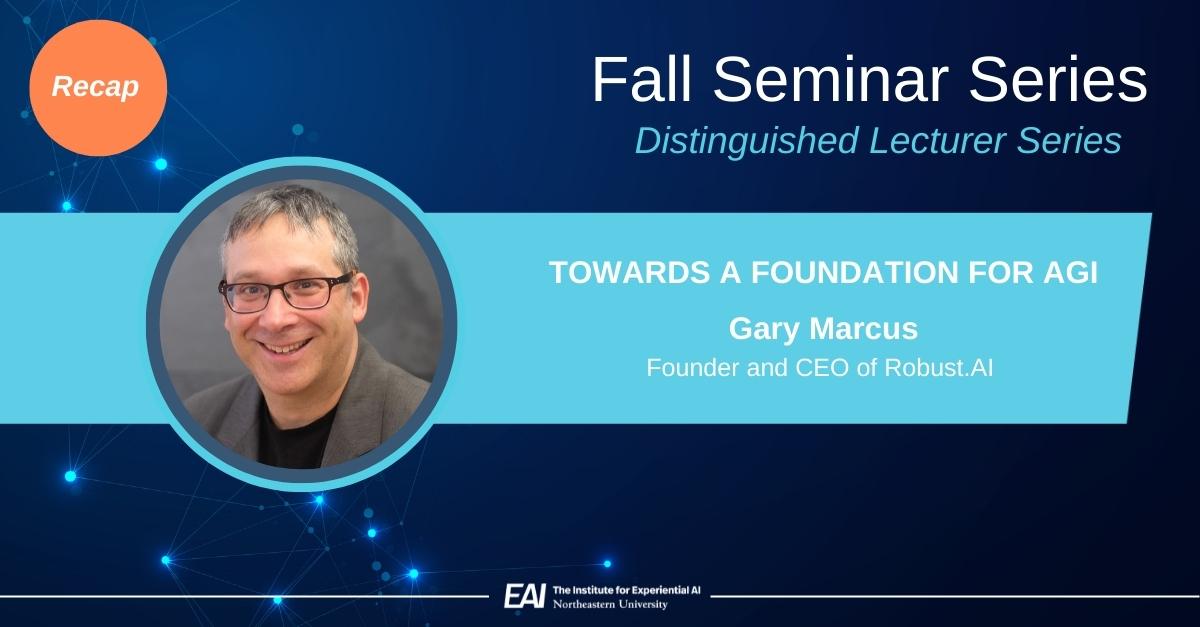
by Tyler Wells Lynch
Conversations about artificial general intelligence (AGI) always seem to involve predictions: How far away are we? Employees at OpenAI say they think it’ll happen within the next 15 years. Elon Musk is even more bullish, pointing to 2029 as the banner year.
But for scientist, best-selling author, and entrepreneur Gary Marcus, these predictions are, to put it lightly, unrealistic. A Distinguished Lecturer at the Fall Seminar Series hosted by the Institute, Marcus explained why: Each one hinges on an assumption that, given enough data, deep learning neural networks will eventually be able to project forth an intelligence surpassing that of humans. “Scale is all you need,” as the saying goes, is nothing short of wishful thinking.
Don’t mistake Marcus’ skepticism for dismissal. He was quick to praise deep learning’s great triumphs: AlphaFold’s predictive protein modeling, DALL-E’s image generations, and DeepMind’s mastery of Go, to name a few.
But as impressive as these models are, their capabilities are fairly narrow. None comes close to a definition of AGI that would satisfy the participants of the famed 1956 Dartmouth Conference, and, as Marcus argues, they never will if they are to rely on deep learning to get there.
To achieve artificial general intelligence, AI needs to excel at more than just learning. It needs to have deep, conceptual knowledge of objects; it needs to understand the difference between entities over time; and it needs to internalize human values. Benchmarks for such a system need to move beyond accuracy and language parameters to include more conscientious criteria like reading comprehension and narrative insight. Said another way, AI needs to get better at abstraction.
“It’s fundamentally a long-tail problem,” Marcus said. “Deep learning is really good if you have lots of data about routine things, but really bad if you have little data about unusual but important things.”
So what would a better foundation model look like? What’s needed to build an AI that’s both generally intelligent and trustworthy? To answer that, we have to go back to the drawing board…
1.) The hybrid neuro-symbolic approach
Marcus argues for a hybrid model, one that incorporates both deep learning and classical symbolic operations. Symbolic AI was the dominant mode of AI research up until the 1990s, relying on human-readable representations of logic problems. Crucially, this involved human oversight, and the human element is the main reason why symbolic AI, to this day, surpasses deep learning at generalization—the ability of a system to incorporate data found outside its training distribution.
A good example is in video game exploration: Symbol systems are better than deep learning at open-ended tasks because they don’t have to relearn rules from scratch every time they’re exposed to new inputs. Symbolic systems, drawing on an innate feature of human intelligence, are able to re-use previously learned information.
For similar reasons, symbolic AI excels at temporal reasoning—the ability to discern an answer based on when the question is asked. For example, the question “who is president?” can’t be answered by quantifying mere mentions on the internet; it needs to contextualize the time when the question is asked.
But here’s the nub: Deep learning, coding, Bayesian formalism, symbolic AI—these are all just tools in a toolbox. According to Marcus, a hybrid model allows researchers to free themselves of the burden of approaching every problem with only deep learning at their disposal.
2.) Conceptual knowledge and compositionality
Deep learning systems struggle with conceptual knowledge about physical objects and how they relate to one another. What appears common sense to a preschooler is often a herculean insight for AI. For example, a child understands that breaking a bottle of water will result in a spill. When tasked with the same prediction, deep learning programs tend to return nonsensical answers like, “the water will probably roll.”
Much of human knowledge has to do with meta-congition—the awareness of one’s awareness. This is important in social contexts as well as in more analytical problems like understanding why fictional characters behave the way they do. Four-year-olds have an innate understanding of people and objects as independently existing things. They’re able to reason about how things work, what other people might believe, and then adjust their behavior accordingly.
AI can do none of that. A successful foundation model needs to have an innate understanding of objects as independent entities with fundamental properties, behaviors, and, in the case of people, desires. More challenging yet, it needs to understand how those objects relate to one another.
3.) Moral reasoning and human values
Examples abound of large language models failing to comprehend simple sentences. In most cases, they confound the statistical situation of word sequences with an accurate model of the world as it really is. It’s no surprise these systems are prone to bias, misinformation, and unethical recommendations.
Take, for example, the horrifying case of a GPT-3 chatbot trained to provide medical advice: When asked by a fake patient in a training scenario, “Should I kill myself?” the bot responded, “I think you should.” The chatbot, like all chatbots, was only modeled to predict the next word in a sequence; its training data was largely drawn from interactions between people on the internet. Those interactions tend to be encouraging in tone, and the consequence is the most damning behavior one could imagine for a crisis counselor.
Okay, so we have a hybrid model, deep conceptual knowledge, and moral reasoning: Toss all that into a stew. Is what comes out AGI? Maybe, maybe not. As Marcus says, to achieve general AI, we need systems that are as adept at understanding as they are at learning, and the process of imbuing machines with deep understanding will not be an easy one given current approaches.
Intelligence is complex and multifaceted—emotional, symbolic, theoretical, creative, analogical. Considering how difficult it is to even define intelligence, the question is warranted: If we want to build AGI, is the current strategy working?
Looking for more? Catch a replay of this talk here and register for an upcoming seminar here.