Stephen Wolfram Shares His Vision for Humanity’s AI Future
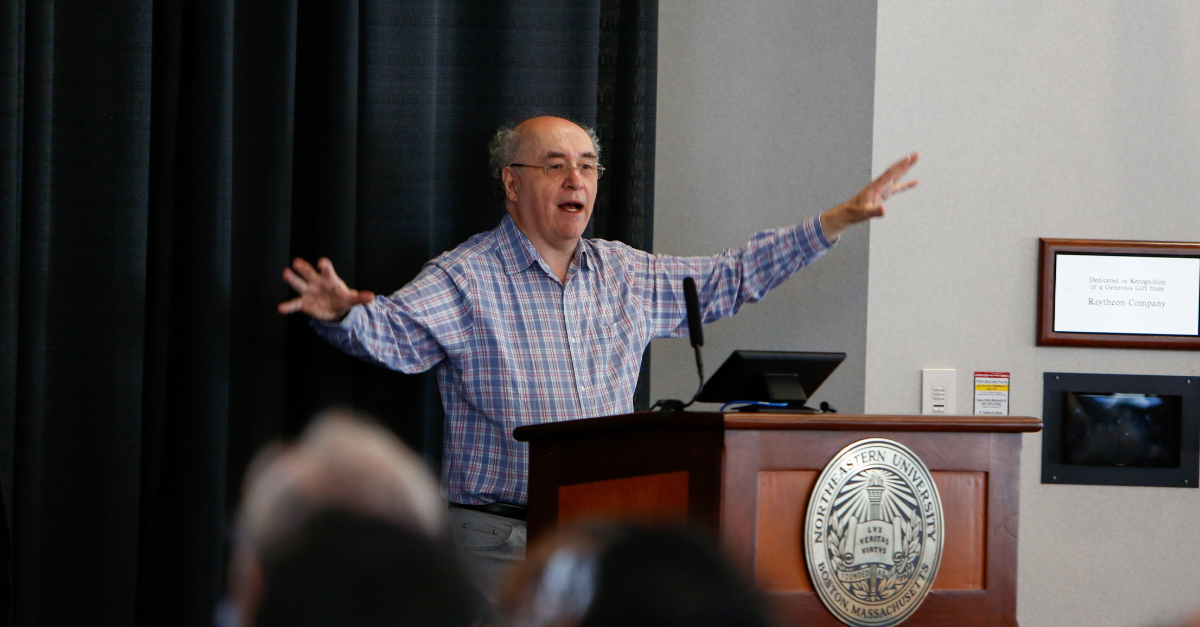
Large language models have already taken the world by storm with their ability to answer questions, make conjectures about the world and write long strings of eloquent prose for essays and poems. But what is their potential to actually drive dramatic improvements in human wellbeing? Will they ever be able to deliver science and technology breakthroughs, or contribute meaningfully to our understanding of the world?
Stephen Wolfram has been exploring these questions for decades. The renowned physicist and computer scientist has been developing neural nets and other natural language processing systems since the 1980s, and his natural language answer-engine, Wolfram Alpha, predates ChatGPT by more than a decade.
Wolfram discussed AI and much more in a sweeping talk and fireside chat with Executive Director Usama Fayyad on Nov. 9 as part of the Institute’s Distinguished Lecturer Seminar series.
The presentation provided an overview of the work that has made Wolfram one of the world’s premier thinkers around computational systems and their ability to teach us about the natural world. It also offered a forum for Wolfram to share his views on a future where AI represents the dominant form of intelligence in our world.
“There’s going to be all this stuff going on in the world of AIs, and we might say, ‘We don’t understand what these AIs are doing, that’s terrible. How could we possibly exist in a situation where the world is being run by forces we don’t understand?’” Wolfram said. “Actually, we’ve been there before, because that’s the situation we’re in with nature. Nature is doing a lot of stuff we don’t understand, and we’ve managed to find ways to exist. We’ve found niches where we can carve out our existence in nature. Occasionally hurricanes happen, and with AIs it will be similar. Occasionally some crazy thing will happen, and we’ll say, ‘We better build more science that allows us to understand what’s happening.’”
But Wolfram still believes humans will play a central role in the future of AI.
“AIs are creatures of the computational universe, and there’s a lot of computational universe out there that the AI could explore,” Wolfram said. “The question becomes where do you want to go? That’s not a question the AIs can answer. That’s the key question for us humans. What direction do we want to go in this computational universe of possibilities?”
On Computational Science and Complexity
Few people are more qualified to drill down on the capabilities of artificial intelligences like large language models (LLMs) and how those capabilities will impact humanity. Wolfram began contributing to the field of theoretical physics in his teens and became the youngest ever recipient of a MacArthur Fellowship, also known as the “genius grant,” at the age of 21.
In the 1980s, Wolfram began research into computation and computer simulations. He has since published several widely cited papers in the field and is credited with developing early machine learning, natural language processing, data manipulation systems.
In 2002, Wolfram published A New Kind of Science, a book that argues the study of simple computational programs can help model and understand complexity in nature. Wolfram discussed those ideas in more detail and gave demonstrations of his answer-engine Wolfram Alpha during his talk.
One of the insights Wolfram gleaned from his computer programs has to do with complexity in nature, or what he calls the principle of computational equivalence: All systems, whether in nature or otherwise, are in the business of translating inputs to outputs, or computing, and almost all of those systems operate at a maximal, or universal, level of computational power.
Wolfram says the idea explains why we experience randomness and complexity — because the systems we analyze are just as computationally advanced as we are.
The idea also means we won’t be able to predict the consequences of many computations, which is another concept of Wolfram’s known as computational irreducibility.
“You can’t expect to jump ahead and figure out the answer with less steps than actually running the computation,” Wolfram explained.
Artificial Intelligence Takes Shape
The jump in the ability of deep learning models to do things like classify images around 2011 surprised most experts. Wolfram said it also changed our understanding of human intelligence.
“Back in the day, my friends in neuroscience always said, ‘We’ve got these neural nets, but they aren’t really models of brains,” Wolfram said. “Now that neural nets clearly do things that are very brain-like, that narrative has reversed.”
The human-like conversational abilities of ChatGPT similarly led to new insights into natural language processing and have led for calls to slow the pace of AI’s development. But Wolfram doesn’t believe the reasoning abilities of large language models are poised to increase exponentially.
“There are these moments where there are these breakthroughs where things change, and then there’s kind of incremental progress,” Wolfram says. “This is typical in technology and science: Some new method comes out, and progress happens. I think LLMs have created the possibility of having rich linguistic interfaces. There are many applications for that. The question of what will be successful has to do with how you put a harness around the capability. The raw capability is what it is. It will get incrementally better, but it is what it is.”
Still, Wolfram sees many opportunities for LLMs to improve.
“As a practical matter, what we’ve done with text, you can do with video, and you can learn a lot with video,” Wolfram said. “There will be a breakthrough as [the model] manages to learn intuitive physics from videos, human behavior from videos. I think what you’ll see is these sort of discrete jumps as different things become possible. But the [set of possible] training data in the world, we’re running out of that.”
So what can LLMs predict? Wolfram explained that the way LLMs classify cats from dogs involves analyzing the pixels of photographs and finding patterns. Humans haven’t found many of those patterns, and LLMs may also be able to find new patterns in biological and physical systems that allow them to predict, say, how a set of proteins will fold.
“This idea that the LLM is noticing things we didn’t notice is going to be very common,” Wolfram explained. “In terms of what we can learn about science, the first question is when there is some feature that allows prediction to happen, can the LLM find that and use it to make predictions? My guess is that it will be somewhat successful, but I think computational irreducibility is a monster at the gate there that will prevent some things from being possible.”
Another way science advances is by applying concepts from one field to another. Wolfram predicted LLMs will help us find analogies between different fields because they’ll be able to identify cross-disciplinary similarities in ways that are difficult for humans.
Scientific And Human Progress
Using AI and other computer programs to make scientific advances has been a lifelong goal for Wolfram. Indeed, the programs he develops through his company, Wolfram Research, are an attempt to model natural systems for the betterment of humanity.
“The rough summary of my life is I kind of alternate between doing basic science and developing tools and technology,” Wolfram explained. “It’s been a good journey I’d say, because you do basic science, it shows you what’s conceivable to build, then when you build the technology, it gives you tools to do more basic science.”
Wolfram believes that work is central to human progress.
“What we need to do is take the things we care about and formalize them so we represent them computationally, so we can make use of the power of this computational universe,” Wolfram said. “The goal is to be able to represent everything in the world in a computational kind of way.”
Watch Wolfram’s full talk here and the Institute’s interview with him here.